
How Companies Use Data to Make Decisions: A Comprehensive Guide
Jan 31, 2025 4 Min Read 3503 Views
(Last Updated)
In today’s data-driven world, companies across industries are increasingly relying on data to inform their decision-making processes. From marketing and product development to operations and strategy, data has become a critical asset that can provide valuable insights, drive innovation, and ultimately lead to better business outcomes.
As the volume and variety of data continue to grow exponentially, companies are recognizing the need to use the power of this invaluable resource. However, collecting and storing data is just the first step; the real challenge lies in transforming raw data into actionable insights that can guide decision-making processes effectively.
In this blog post, we will explore how companies use data to make informed decisions across various aspects of their operations. We’ll learn the different types of data companies collect, the analytical techniques they employ, and the specific decision-making areas where data plays an important role.
Table of contents
- Types of Data, Companies Collect
- How Companies Use Data to Make Decisions
- Marketing and Customer Analytics
- Product Development and Innovation
- Sales and Revenue Management
- Supply Chain and Logistics
- Operations and Process Optimization
- Finance and Risk Management
- Human Resources
- Strategic Planning and Decision Support
- Conclusion
- FAQs
- How do companies determine which data is most relevant for making strategic decisions?
- What role do data analytics tools play in helping companies interpret and derive insights from their data?
- How can companies ensure data accuracy and reliability?
Types of Data, Companies Collect
Before we learn how companies use data for decision-making, it’s essential to understand the different types of data they collect. Generally, data can be categorized into three main types:
- Structured Data: This type of data is highly organized and follows a predefined format, making it easy to store and analyze. Examples include numerical data in spreadsheets, customer records in databases, and financial transactions.
- Unstructured Data: Unstructured data is more complex and lacks a specific format or structure. It includes text data from social media posts, emails, and customer reviews, as well as multimedia data such as images, videos, and audio files.
- Semi-structured Data: As the name suggests, semi-structured data falls somewhere between structured and unstructured data. It has some organizational properties but lacks the strict formatting of structured data. Examples include XML files, JSON data, and log files.
Companies often collect data from various sources, including their internal systems (e.g., sales records, customer databases, inventory management systems), external sources (e.g., market research, social media, publicly available datasets), and Internet of Things (IoT) devices.
Ready to elevate your data science skills? Enroll now in the IITK Data Science Professional Certification course and unlock a world of opportunities in machine learning and predictive analytics!
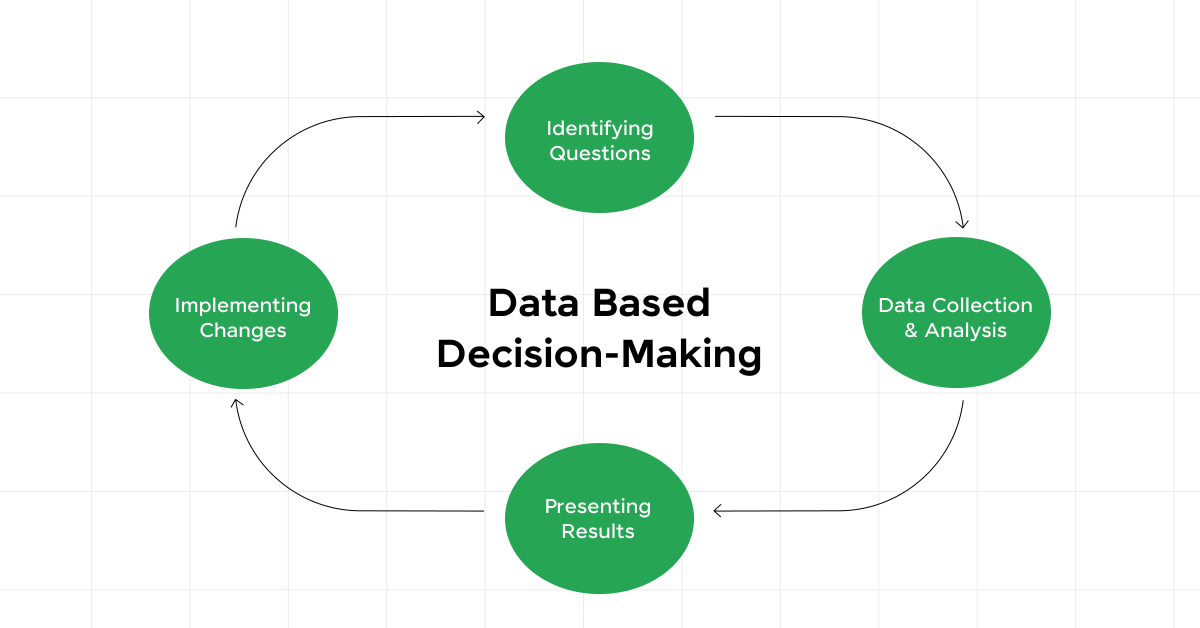
Having learned the types of data companies gather, it’s time to explore how this data becomes the cornerstone of decision-making. Let’s explore how companies use data to inform and drive their strategic choices.
How Companies Use Data to Make Decisions
Here’s an overview of how companies use data to make decisions across various aspects of their operations:
1. Marketing and Customer Analytics
Companies use customer data, market research, and analytics to optimize their marketing efforts and enhance customer experiences. This includes customer segmentation, campaign optimization, behavior analysis, churn prevention, sentiment analysis, and personalized recommendations.
By analyzing data, companies can better understand customer needs, preferences, and behaviors, enabling more targeted and effective marketing strategies.
- Customer segmentation and targeting
- Campaign optimization and measurement
- Customer behavior and preference analysis
- Predictive modeling for churn prevention
- Sentiment analysis from social media and reviews
- Personalization and recommendation engines
Also Read: 12 Real-World Data Science Examples: Power Of Data Science
2. Product Development and Innovation
Data plays an important role in driving product innovation and development. Companies analyze market trends, user feedback, product usage data, and A/B testing results to identify new opportunities, refine existing products, and ensure they meet customer demands. Predictive maintenance and quality control analytics help improve product reliability and performance.
- Market research and trend analysis
- Product usage analytics and feature adoption
- A/B testing and product experimentation
- Predictive maintenance and quality control
- Demand forecasting and inventory optimization
Also Read: 9 Amazing Real-World Data Engineering Applications Around Us!
3. Sales and Revenue Management
Data-driven insights support sales and revenue management processes, including lead scoring, sales forecasting, pricing optimization, customer lifetime value analysis, and cross-selling/upselling recommendations. By using data, companies can prioritize high-value leads, forecast demand accurately, and maximize revenue through personalized offerings and promotions.
- Lead scoring and prioritization
- Sales forecasting and pipeline management
- Pricing optimization and promotion strategies
- Customer Lifetime Value Analysis
- Cross-selling and upselling recommendations
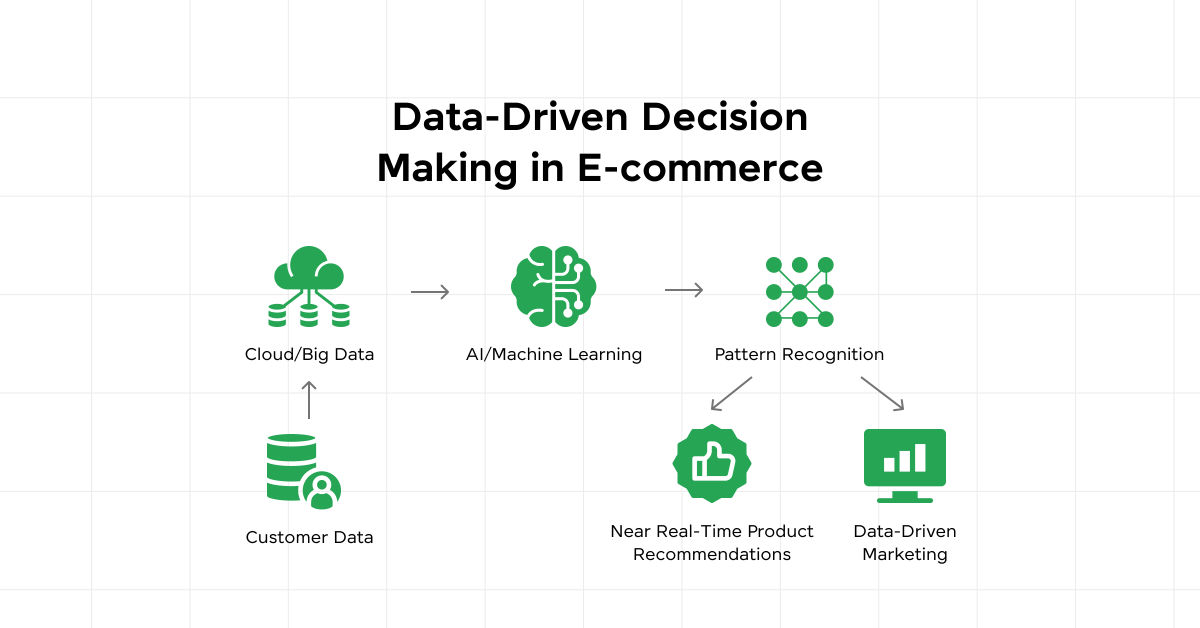
Also Read: Best Way to Learn Data Engineering in 2025
4. Supply Chain and Logistics
Companies use data to optimize their supply chain and logistics operations, including inventory management, demand planning, route optimization, supplier risk assessment, predictive maintenance, and real-time tracking. Data-driven insights help minimize costs, reduce waste, improve efficiency, and ensure timely delivery of goods and services.
- Inventory management and demand planning
- Route optimization and fleet management
- Supplier risk assessment and evaluation
- Predictive maintenance for equipment and machinery
- Real-time tracking and visibility across the supply chain
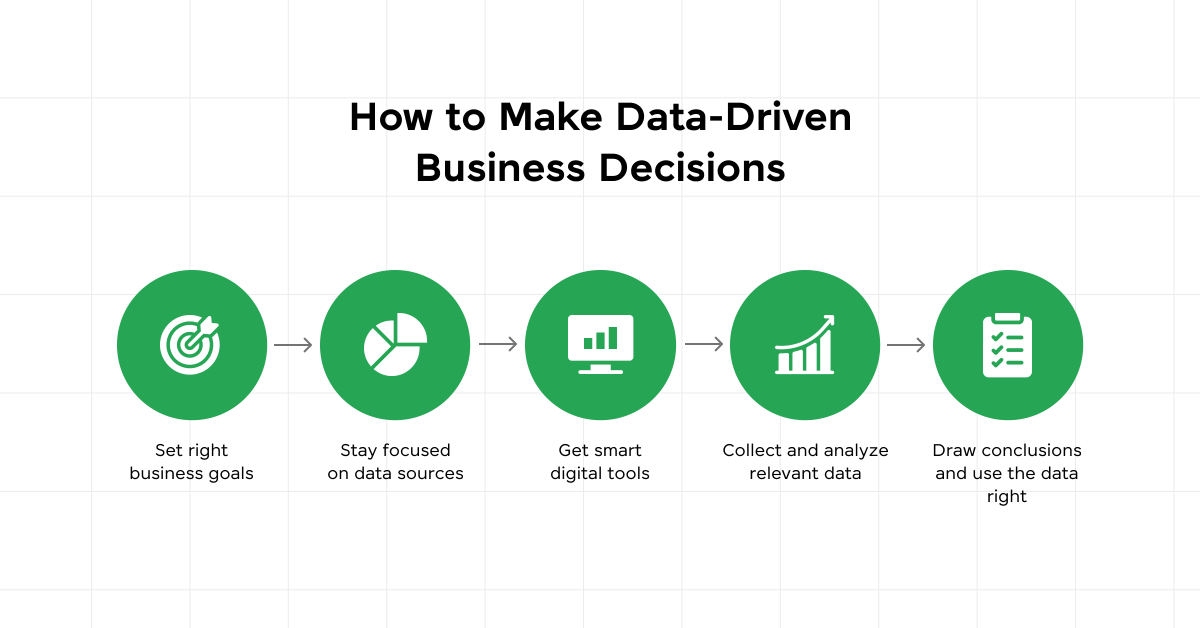
Also Read: 9 Most Creative Data Engineering Project Ideas To Kickstart Your Career
5. Operations and Process Optimization
Data analytics supports various operational processes, such as predictive maintenance, production line optimization, resource allocation, process mining, and sustainability analytics. By analyzing operational data, companies can identify bottlenecks, minimize downtime, optimize resource utilization, and improve overall efficiency and productivity.
- Predictive maintenance for equipment and assets
- Production line optimization and quality control
- Resource allocation and workforce planning
- Process mining and bottleneck identification
- Energy consumption and sustainability analytics
Also Read: Data Engineering Syllabus 2025 | A Complete Guide
6. Finance and Risk Management
Financial institutions and companies use data to support financial forecasting, budgeting, credit risk assessment, fraud detection, investment portfolio optimization, compliance monitoring, and risk modeling. Data-driven insights help mitigate financial risks, ensure regulatory compliance, and make informed investment and financial decisions.
- Financial forecasting and budgeting
- Credit risk assessment and fraud detection
- Investment portfolio optimization
- Compliance monitoring and regulatory reporting
- Risk modeling and scenario analysis
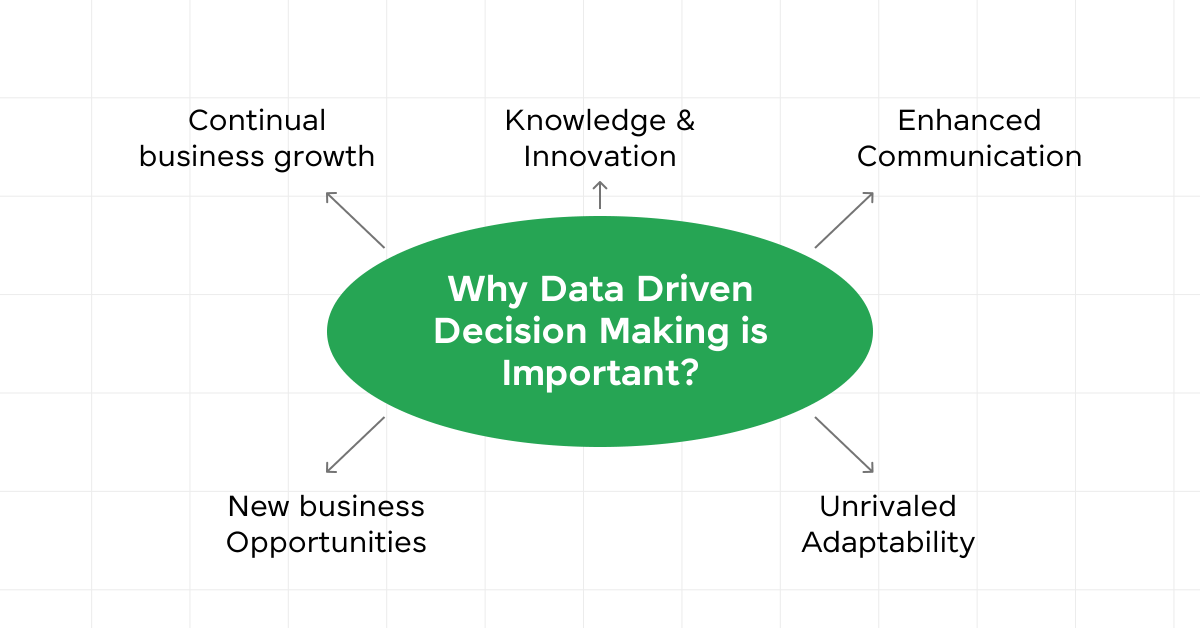
Also Read: 10 Best Data Science Online Courses for Beginners | 2025
7. Human Resources
HR departments use data for talent acquisition, employee engagement and retention analysis, workforce planning, performance management, and learning program optimization. Data-driven insights help companies attract and retain top talent, identify skill gaps, and foster a productive and engaged workforce.
- Talent acquisition and recruitment analytics
- Employee engagement and retention analysis
- Workforce planning and skill gap analysis
- Performance management and productivity metrics
- Learning and development program optimization
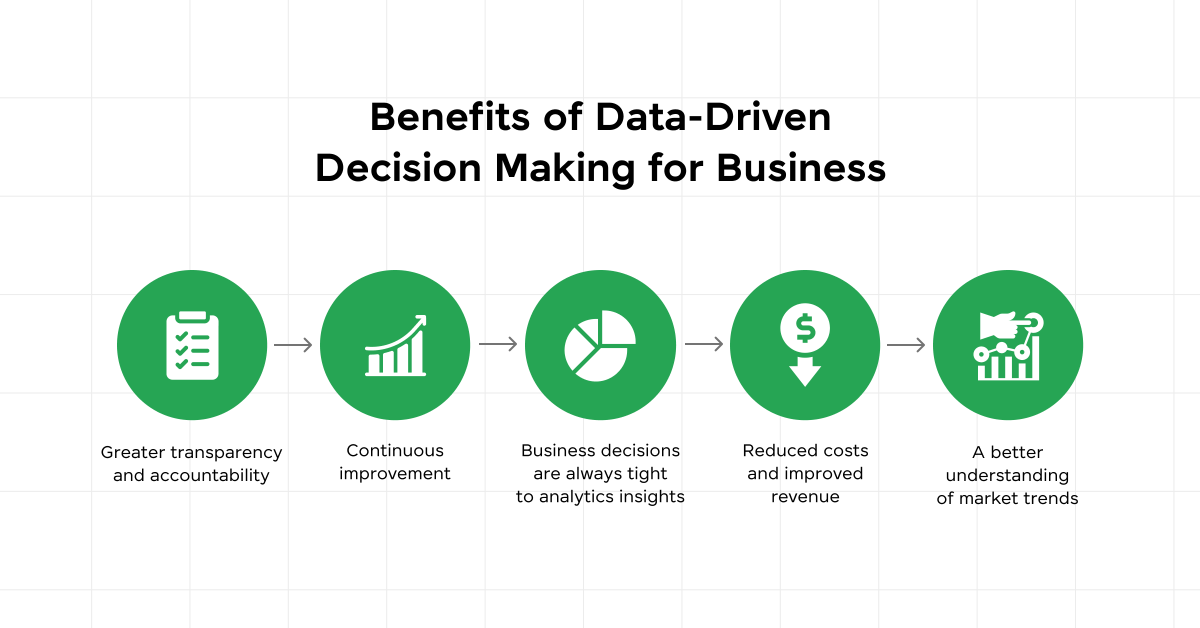
8. Strategic Planning and Decision Support
At the strategic level, companies use data for market and competitive intelligence, merger and acquisition target evaluation, business model simulations, resource allocation, and KPI tracking. Data-driven insights support informed decision-making, strategic planning, and the identification of growth opportunities.
- Market and competitive intelligence
- Merger and acquisition target evaluation
- Business model and pricing strategy simulations
- Resource allocation and investment prioritization
- Key performance indicator (KPI) tracking and dashboards
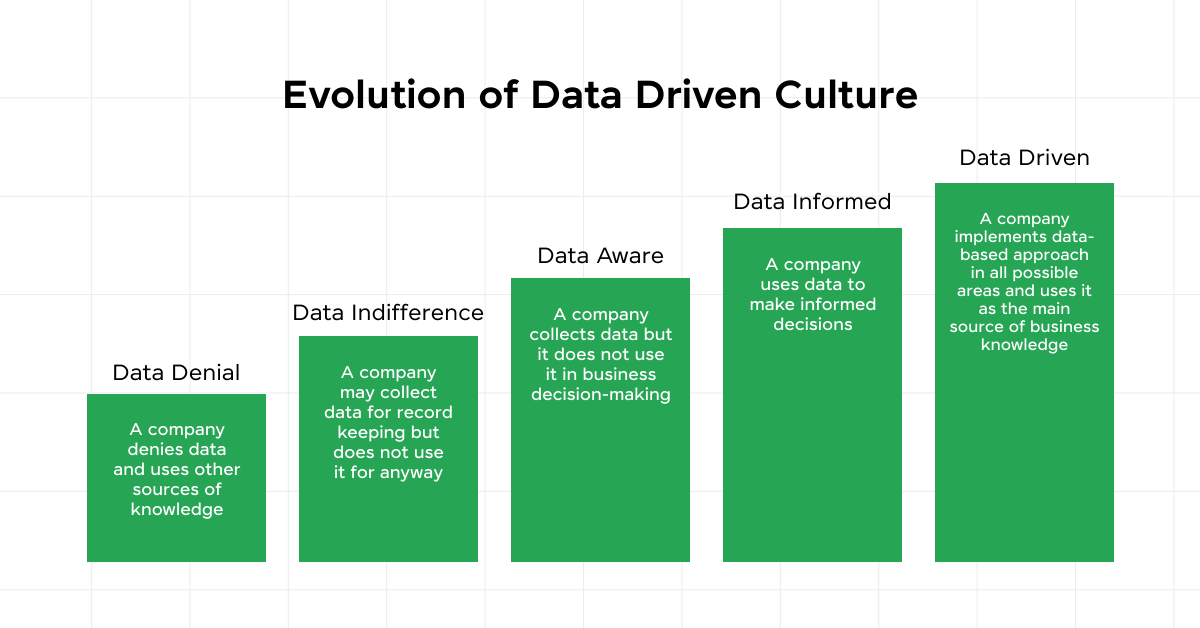
Across these various domains, companies use various data analytics techniques, including descriptive, diagnostic, predictive, and prescriptive analytics. They also employ data visualization, machine learning, and artificial intelligence to derive insights and support decision-making processes.
Also Read: Scope of Data Engineering in India in 2025
Effective data-driven decision-making requires a strong data culture, robust data governance, and the right mix of technology, processes, and skilled personnel. Companies that can successfully use the power of data will gain a competitive advantage, drive innovation, and make informed choices aligned with their strategic goals and customer needs.
Don’t miss out on the chance to become a Certified Data Science Course with IITK’s comprehensive program. Take the first step towards mastering essential skills in data analysis and machine learning. Enroll today!
Conclusion
As companies continue to generate and collect vast amounts of data, the ability to effectively use this valuable resource will become increasingly critical for success. By embracing data-driven decision-making, companies can gain a competitive edge, drive innovation, and make informed choices that align with their strategic goals and customer needs.
Remember, data alone is not enough – it’s the ability to transform data into actionable insights and integrate those insights into decision-making processes that truly unlock the power of data.
By following best practices, addressing challenges, and fostering a data-driven culture, companies can learn the complexities of data-driven decision-making and position themselves for long-term success in an increasingly data-driven world.
Also, Explore: Best Practices for Data Quality Assurance in Data Engineering [2025]
FAQs
Companies prioritize data aligned with strategic objectives and KPIs, focusing on metrics like sales, customer feedback, and market trends.
Data analytics tools enable analysis ranging from basic spreadsheets to advanced platforms with machine learning. They uncover patterns and trends, supporting decision-making with descriptive, diagnostic, predictive, and prescriptive analytics.
Companies ensure data accuracy through validation processes, regular audits, governance frameworks, collaboration between stakeholders, and the use of quality tools to monitor compliance and integrity.
Did you enjoy this article?