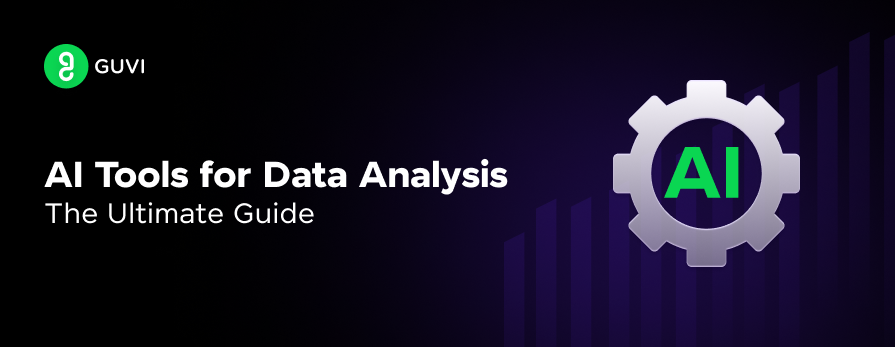
AI Tools for Data Analysis: The Ultimate Guide
Oct 22, 2024 5 Min Read 1502 Views
(Last Updated)
The world is based on data these days. We can derive whatever information we need if we have sufficient data. The role that analyzes the data and comes to conclusions is data analysis.
Since AI is taking the world by storm, its influence is present in the field of data analysis as well. That’s why the article will cover the best AI tools for data analysis.
By learning about these AI tools for data analysis you equip yourself with the knowledge of invaluable tools that can make your workflow more efficient. So, without any delay, let us get started!
Table of contents
- Top 10 AI Tools for Data Analysis – Overview
- Best AI Tools for Data Analysis
- DataRobot
- H2O.ai
- Google Cloud AutoML
- IBM Watson Studio
- Microsoft Azure Machine Learning
- Alteryx
- KNIME
- RapidMiner
- Tableau
- Amazon SageMaker
- Conclusion
- FAQs
- What are the best AI tools for data analysis?
- How can AI tools improve the data analysis process?
- What factors should be considered when choosing an AI tool for data analysis?
- Which AI tools are most suitable for beginners in data analysis?
- How do AI tools integrate with existing data analysis workflows?
- What are the limitations of using AI tools for data analysis?
- Can AI tools for data analysis be customized to specific industry needs?
Top 10 AI Tools for Data Analysis – Overview
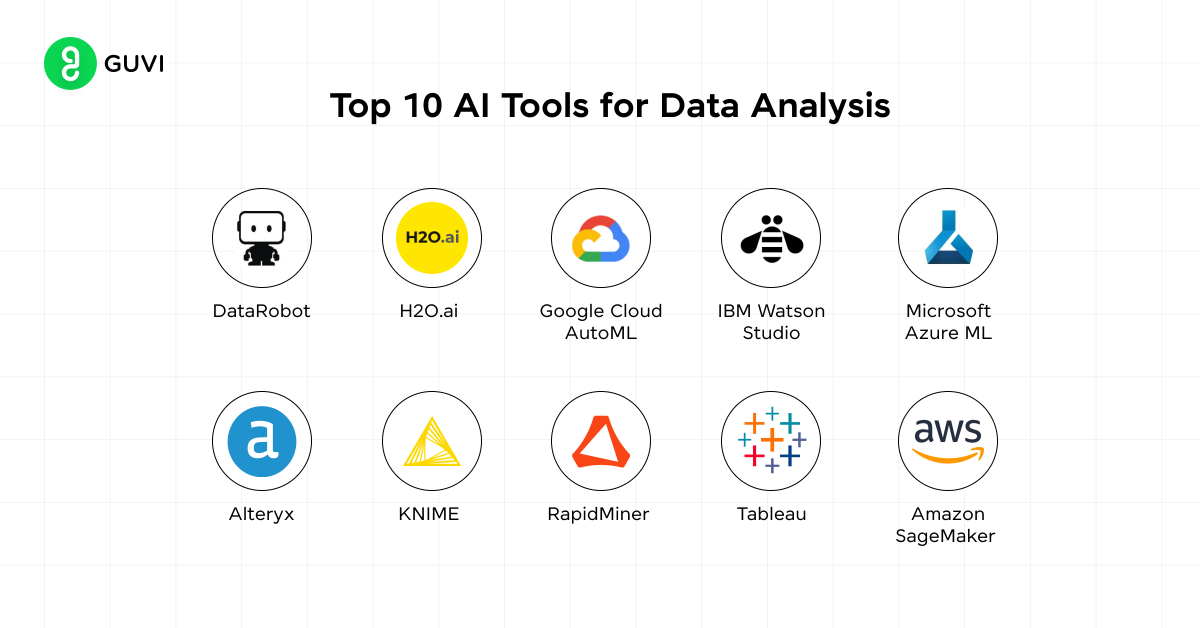
Here’s an overview of the top 10 AI tools for data analysis:
S.No. | Tool Name | Features | Compatibility | Ease of Integration | Access Now |
1 | DataRobot | Automated ML, Data Prep, Model Deployment | Windows, macOS, Linux | Easy | Access DataRobot |
2 | H2O.ai | Open-source ML, AutoML, Deep Learning | Windows, macOS, Linux | Moderate | Access H2O.ai |
3 | Google Cloud AutoML | Natural Language, Vision, Translation | Cloud-based | Easy | Access Google AutoML |
4 | IBM Watson Studio | Data Analysis, Visualizations, ML | Windows, macOS, Cloud | Moderate | Access Watson Studio |
5 | Microsoft Azure ML | Predictive Analytics, Model Training | Cloud-based | Easy | Access Azure ML |
6 | Alteryx | Data Wrangling, Automation, Visualizations | Windows, macOS | Easy | Access Alteryx |
7 | KNIME | Data Prep, ML, Deep Learning | Windows, macOS, Linux | Moderate | Access KNIME |
8 | RapidMiner | Data Science, Predictive Analytics | Windows, macOS, Linux | Easy | Access RapidMiner |
9 | Tableau | Data Visualizations, Predictive Modeling | Windows, macOS, Cloud | Easy | Access Tableau |
10 | Amazon SageMaker | Model Training, Deployment, Data Prep | Cloud-based | Easy | Access SageMaker |
Best AI Tools for Data Analysis
We have seen the overview of the top 10 AI tools for data analysis, now let us have an in-depth look at these phenomenal tools!
1. DataRobot
DataRobot is an automated machine learning platform that accelerates the process of building and deploying AI models. It’s perfect for users who want to streamline the data analysis process without coding expertise, offering robust tools for data preparation and model deployment.
Core Features:
- Automated machine learning
- Data preparation and cleaning tools
- Model deployment and monitoring
Compatibility: Works across Windows, macOS, and Linux.
Ease of Use: Very beginner-friendly with drag-and-drop functionality.
Scalability: It is suitable for small businesses and large enterprises.
Support for Data Formats: CSV, JSON, SQL, and more.
Visualization Capabilities: Built-in data visualizations for model results.
Performance Metrics: High speed for processing large datasets and strong predictive accuracy.
Security: Enterprise-grade security with data encryption and user authentication.
Use Case: Frequently used in financial services for fraud detection, as it quickly processes transactional data to detect anomalies.
User Reviews and Ratings: 4.6 / 5 (Source: Gartner)
Pricing: Contact them for custom pricing based on needs.
Try Now: Website Link
2. H2O.ai
H2O.ai is an open-source platform that excels in AutoML and deep learning. Its flexibility and scalability make it a popular choice for data scientists who want to customize their machine-learning workflows while benefiting from advanced features like deep learning and AI capabilities.
Core Features:
- Open-source machine learning
- AutoML and deep learning support
Compatibility: Compatible across Windows, macOS, Linux, and cloud environments.
Ease of Use: Moderate—suitable for those with some ML experience.
Scalability: Scalable for enterprise-level tasks.
Support for Data Formats: CSV, JSON, SQL, and more.
Visualization Capabilities: Extensive visualization options for model analysis.
Performance Metrics: High-performing with large datasets and complex models.
Security: Provides encryption and compliance with GDPR.
Use Case: Commonly used in healthcare for patient data analysis and predictive health diagnostics.
User Reviews and Ratings: 4.4 / 5 (Source: Gartner)
Pricing: Contact them for pricing and you can request a demo if you want as well.
Try Now: Website Link
3. Google Cloud AutoML
Google Cloud AutoML enables users to create custom machine-learning models without the need for extensive coding knowledge.
With features like natural language processing and image recognition, it is ideal for businesses looking to integrate AI into their operations easily.
Core Features:
- Natural language processing (NLP)
- Image and video analysis
- Translation models
Compatibility: Cloud-based, accessible via any device.
Ease of Use: Very easy—ideal for users without extensive coding experience.
Scalability: Fully scalable on Google’s cloud infrastructure.
Support for Data Formats: Text, images, and videos.
Visualization Capabilities: Strong visualizations for model insights.
Performance Metrics: High accuracy in language, image, and video processing.
Security: Google-grade security with data protection and user access control.
Use Case: Widely used in e-commerce for product recommendation systems and image-based searches.
User Reviews and Ratings: 4.4 / 5 (Source: Gartner)
Pricing: Pay-as-you-go model that starts from 11800 INR.
Try Now: Website Link
4. IBM Watson Studio
IBM Watson Studio offers a collaborative environment where teams can build, train, and deploy AI models. It’s designed for enterprises looking to harness the power of AI for large-scale data projects while enabling seamless teamwork and model management.
Core Features:
- AI model development
- Data preparation and collaborative workspace
Compatibility: Windows, macOS, and cloud environments.
Ease of Use: Moderate—best suited for intermediate users who know the basics of data science and data analysis.
Scalability: Ideal for large enterprises with big data projects.
Support for Data Formats: JSON, SQL, CSV, and more.
Visualization Capabilities: Interactive visualizations for data and model insights.
Performance Metrics: Excellent for large-scale AI and data projects.
Security: Enterprise-grade security with GDPR compliance and advanced data encryption.
Use Case: Commonly used in banking for analyzing large transaction datasets and providing personalized customer insights.
User Reviews and Ratings: 4.2 / 5 (Source: G2)
Pricing: It offers both free tier and professional model. The professional model follows a pay-as-you-go approach that starts from 84 INR/hour.
Try Now: Website Link
5. Microsoft Azure Machine Learning
Azure Machine Learning provides a comprehensive platform for developing and deploying machine learning models.
It integrates seamlessly with other Microsoft services, making it a go-to for businesses already using Azure for data storage and computing.
Core Features:
- Predictive analytics
- End-to-end AI model building
Compatibility: Cloud-based, integrates with Microsoft’s Azure services.
Ease of Use: Simple to moderate, depending on familiarity with Azure.
Scalability: Highly scalable, suitable for both small businesses and large enterprises.
Support for Data Formats: Supports common formats like CSV, JSON, and SQL.
Visualization Capabilities: Great visualization tools for monitoring and evaluating models.
Performance Metrics: High-performance for building and deploying predictive models.
Security: Strong security measures, including data encryption, compliance with GDPR, and user authentication.
Use Case: Often used in manufacturing for predictive maintenance and optimizing production workflows.
User Reviews and Ratings: 4.2 / 5 (Source: Gartner)
Pricing: Contact for custom pricing based on needs.
Try Now: Website Link
6. Alteryx
Alteryx is known for its user-friendly data preparation and automation tools. It allows users to quickly clean, manipulate, and analyze data with minimal coding, making it ideal for marketers, analysts, and data-driven teams.
Core Features:
- Data wrangling and automation
- Extensive visualizations
Compatibility: Works on Windows and macOS.
Ease of Use: Easy—ideal for users with minimal coding experience.
Scalability: Suitable for small to mid-sized teams and companies.
Support for Data Formats: Supports CSV, Excel, SQL, and more.
Visualization Capabilities: Rich visualizations for data insights and workflows.
Performance Metrics: Fast data processing and efficient automation for large datasets.
Security: Enterprise-grade security features.
Use Case: Used in marketing for customer segmentation and campaign optimization based on data-driven insights.
User Reviews and Ratings: 4.6 / 5 (Source: G2)
Pricing: Annual subscription model of 4,35,900 INR/month.
Try Now: Website Link
7. KNIME
KNIME is an open-source platform that focuses on data integration, processing, and machine learning. It’s widely used in research and industrial applications, offering users a flexible environment for complex data workflows and deep learning projects.
Core Features:
- Data preparation and mining
- Machine learning and deep learning integration
Compatibility: Windows, macOS, Linux.
Ease of Use: Moderate—designed for users with some data science knowledge.
Scalability: Great for scaling small projects to enterprise level.
Support for Data Formats: Supports CSV, JSON, and many other formats.
Visualization Capabilities: Robust visualizations for analyzing complex data workflows.
Performance Metrics: Good performance with data wrangling and integration tasks.
Security: Secure with enterprise-level data protection features.
Use Case: Popular in pharmaceuticals for analyzing clinical trial data and improving drug development efficiency.
User Reviews and Ratings: 4.8 / 5 (Source: Gartner)
Pricing: It has community and business options where the community has both free and a team plan of 99 USD/month. The business option starts from 334800 INR/year
Try Now: Website Link
8. RapidMiner
RapidMiner is a powerful data science platform that supports the complete workflow of data preparation, model building, and deployment.
It’s especially useful for organizations needing predictive analytics, thanks to its easy-to-use, drag-and-drop interface.
Core Features:
- Predictive analytics
- Data science workflows
Compatibility: Works across Windows, macOS, and Linux.
Ease of Use: Easy to moderate—offers drag-and-drop functionality for non-coders.
Scalability: Scalable for mid to large businesses.
Support for Data Formats: Supports a wide range of data formats including CSV, JSON, and SQL.
Visualization Capabilities: Extensive visualization tools for building and analyzing models.
Performance Metrics: Strong in terms of speed and handling large datasets.
Security: Enterprise-level security with GDPR compliance.
Use Case: Commonly used in telecommunications to predict customer churn and optimize network services.
User Reviews and Ratings: 4.6 / 5 (Source: G2)
Pricing: Contact them for pricing details.
Try Now: Website Link
9. Tableau
Tableau, enhanced with AI features, is one of the best tools for visualizing and understanding complex data.
Its intuitive interface combined with AI-driven insights makes it a favorite for businesses that rely on data visualization for decision-making.
Core Features:
- Data visualizations
- Predictive modeling using AI
Compatibility: Works across Windows, macOS, and the cloud.
Ease of Use: Very easy—one of the best visualization tools available.
Scalability: Scalable for both small teams and large organizations.
Support for Data Formats: Supports CSV, Excel, SQL, and other data formats.
Visualization Capabilities: Industry-leading visualizations with AI-driven insights.
Performance Metrics: Strong for data visualization but requires integrations for heavy ML tasks.
Security: Offers encryption and role-based access controls.
Use Case: Widely used in retail for sales data analysis, inventory optimization, and customer behavior insights.
User Reviews and Ratings: 4.4 / 5 (Source: G2)
Pricing: Contact for custom pricing based on needs.
Try Now: Website Link
10. Amazon SageMaker
Amazon SageMaker is a cloud-based machine-learning platform designed to help developers build, train, and deploy machine-learning models quickly.
Built on AWS, it’s perfect for users looking to scale their AI operations with enterprise-level infrastructure.
Core Features:
- Model training and deployment
- Data wrangling and preparation
Compatibility: Cloud-based, built on AWS.
Ease of Use: Moderate—ideal for developers familiar with AWS.
Scalability: Highly scalable for enterprise-level data projects.
Support for Data Formats: Supports various formats such as CSV, JSON, SQL.
Visualization Capabilities: Offers basic visualization tools for tracking model performance.
Performance Metrics: High-performing for training large-scale models with AWS infrastructure.
Security: AWS-level security with encryption and access management.
Use Case: Amazon SageMaker is widely used in e-commerce and retail for recommendation engines.
User Reviews and Ratings: 4.3 / 5 (Source: Gartner)
Pricing: You have to request a pricing quote.
Try Now: Website Link
With this, we’ll come to our long list of 10 best AI tools for data analysis. We hope you find comfortable with these tools and make your workflow smooth and efficient!
If you want to learn more about Data Science and how it enhances your career profile, consider enrolling for GUVI’s Data Science Course which teaches everything you need and will also provide an industry-grade certificate!
Conclusion
In conclusion, choosing the right AI tool for data analysis can make all the difference in your work. Whether you’re looking for automation, scalability, or better visualizations, the best AI tools for data analysis offer a wide range of features that can meet your needs.
Consider your current workflow, compatibility with existing platforms, and your data format requirements before making a choice.
FAQs
1. What are the best AI tools for data analysis?
Some of the best AI tools include DataRobot, H2O.ai, Google Cloud AutoML, IBM Watson Studio, and Microsoft Azure ML.
2. How can AI tools improve the data analysis process?
AI tools automate complex tasks, reduce human errors, and provide faster, more accurate insights, allowing you to focus on making data-driven decisions.
3. What factors should be considered when choosing an AI tool for data analysis?
Key factors include ease of use, compatibility, scalability, data format support, visualization capabilities, and security.
4. Which AI tools are most suitable for beginners in data analysis?
Google Cloud AutoML and DataRobot are excellent for beginners, thanks to their easy interfaces and automated processes.
5. How do AI tools integrate with existing data analysis workflows?
Most AI tools are designed to integrate with existing platforms like cloud environments, databases, and other data analysis tools.
6. What are the limitations of using AI tools for data analysis?
AI tools may require high initial setup costs, depend on large datasets for accuracy, and sometimes lack flexibility for very niche use cases.
7. Can AI tools for data analysis be customized to specific industry needs?
Yes, many AI tools allow customization and offer features tailored to specific industries, such as healthcare, finance, and marketing.
Did you enjoy this article?