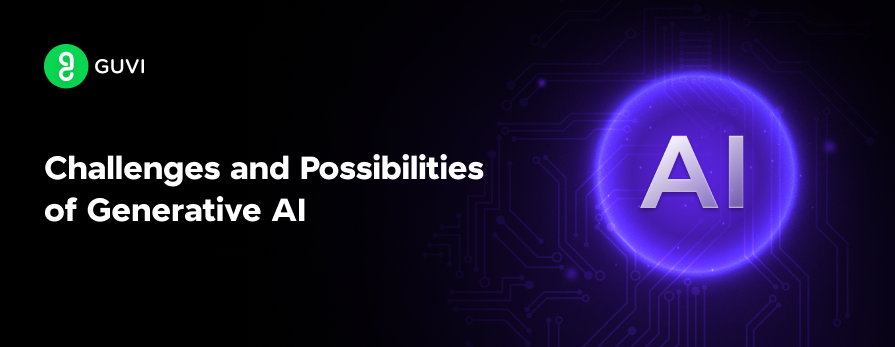
Challenges and Possibilities of Generative AI
Oct 22, 2024 5 Min Read 3677 Views
(Last Updated)
Generative AI has emerged in recent years, capturing the public imagination and raising both excitement and concern about its potential impacts. These powerful systems can generate text, images, audio, video, and other content that in many cases is nearly indistinguishable from human-created work.
The rapid advances in generative AI capabilities have opened up many possibilities, but also present significant challenges that need to be grappled with. In this post, we’ll explore some of the key opportunities and risks associated with generative AI as it continues to evolve and proliferate.
Table of contents
- What is Generative AI?
- Challenges and Possibilities of Generative AI
- Data Privacy and Security
- Bias in AI Models
- Ethical Concerns
- Lack of Strategic Roadmap
- Scalability Issues
- Lack of Skilled Workforce
- High Computational Costs
- Quality Control
- Integration with Existing Systems
- Governance and Regulatory Compliance
- Looking Ahead: The Future of Generative AI
- Conclusion
- FAQs
- What ethical concerns are associated with Generative AI?
- How does Generative AI impact productivity and creativity?
- What challenges exist in ensuring reliable and high-quality Generative AI outputs?
- How can Generative AI be used responsibly?
- What are the future trends in Generative AI?
What is Generative AI?
Generative AI is a type of artificial intelligence that creates new content or data that resembles the input it has been trained on. Unlike traditional AI systems, which might classify data or make predictions, generative AI models can produce original outputs such as text, images, music, and even code. These systems learn patterns and structures from vast amounts of data and then use this knowledge to generate new, similar content.
Examples of generative AI include:
- Text generation models like GPT (Generative Pre-trained Transformer)
- Image synthesis models like DALL-E
- Music composition tools
Generative AI has applications in various fields:
- Creative arts
- Content creation
- Design
- Scientific research
It helps:
- Automate tasks
- Inspire innovation
- Enhance human creativity

If you’re ready to learn AI and take your skills to the next level, enroll in GUVI’s ChatGPT course today!
Challenges and Possibilities of Generative AI
Generative AI is gaining momentum across industries, with 67% of senior IT leaders planning to prioritize it within the next 18 months, and one-third of them identifying it as their top priority. While the potential of this technology is vast, it comes with significant challenges that must be addressed to fully leverage its capabilities. Below are ten key challenges and their corresponding possible solutions.
1. Data Privacy and Security
Challenge
Generative AI systems require massive datasets, which raises concerns about data privacy and security. Sensitive information could be exposed or misused during the AI training process.
Possible Solution
Implement robust data anonymization techniques and encryption methods to protect sensitive information. Additionally, adhering to strict data governance policies and ensuring compliance with regulations such as GDPR can help mitigate privacy risks. As over 43% of executive leaders are currently testing generative AI tools, ensuring data privacy is critical during this experimental phase.

2. Bias in AI Models
Challenge
AI models can perpetuate and even amplify biases present in the training data, leading to unfair and discriminatory outcomes.
Possible Solution
Incorporate diverse datasets during training to minimize biases. Regularly audit AI models for bias and implement fairness checks. Also, involve interdisciplinary teams, including ethicists, in the development process to ensure ethical considerations are addressed. This approach is essential given that more than 70% of executives are hesitant to fully adopt generative AI due to concerns like bias.

3. Ethical Concerns
Challenge
The creation of deepfakes and misinformation using generative AI poses significant ethical challenges, as it can be used maliciously to deceive or harm individuals.
Possible Solution
Develop and enforce strict ethical guidelines and policies for the use of generative AI. Promote transparency by clearly labeling AI-generated content and implementing technologies that can detect and flag deepfakes. Establishing these ethical boundaries is crucial as generative AI’s role expands, particularly in media and content creation.
If you want to explore GenAI in-depth and transform your ideas into reality, enroll in GUVI’s Generative AI course today!
4. Lack of Strategic Roadmap
Challenge
According to a BCG report, over 80% of participants identified the absence of a strategic roadmap as a major obstacle to implementing generative AI effectively.
Possible Solution
Organizations should develop a clear, long-term strategic roadmap for AI adoption, including setting measurable goals, identifying key use cases, and allocating resources effectively. Involve senior leadership in the planning process to ensure alignment with business objectives. This strategic planning will be vital as companies move from testing phases to full implementation.
5. Scalability Issues
Challenge
Scaling generative AI models to handle large volumes of data and complex tasks can be challenging, especially for smaller organizations.
Possible Solution
Utilize cloud-based AI platforms that offer scalable resources and services. Additionally, implement modular AI frameworks that allow for incremental scaling as the organization’s needs grow. As generative AI adoption increases, scalable solutions will be key to maintaining competitive advantages.
6. Lack of Skilled Workforce
Challenge
A shortage of skilled professionals with expertise in AI and machine learning hinders the effective deployment of generative AI.
Possible Solution
Invest in training and upskilling existing employees in AI and related fields. Collaborate with educational institutions to develop AI-specific programs and certifications. Additionally, consider hiring specialized AI talent or partnering with AI-focused firms. This investment in human capital is crucial, as the potential productivity gains from generative AI could boost global GDP by 7% over the next decade, according to a July 2023 report by Goldman Sachs.

7. High Computational Costs
Challenge
Training and running generative AI models require substantial computational power, leading to high costs.
Possible Solution
Optimize AI models to be more efficient in terms of computational resources. Leverage cloud computing and AI-as-a-Service (AIaaS) solutions to reduce the financial burden. Additionally, consider using more energy-efficient hardware and algorithms to manage costs better while scaling AI initiatives.
8. Quality Control
Challenge
Ensuring the reliability and accuracy of AI-generated content is challenging, as models can sometimes produce incorrect or nonsensical outputs (hallucinations).
Possible Solution
Implement robust validation and quality control processes, including human-in-the-loop systems where humans review and approve AI-generated content. Regularly update models with fresh data to improve accuracy and reduce errors. Maintaining high-quality outputs is essential as companies move towards broader adoption and implementation of generative AI systems.
9. Integration with Existing Systems
Challenge
Integrating generative AI with legacy systems and workflows can be complex and time-consuming.
Possible Solution
Adopt a phased integration approach, starting with pilot projects to identify potential challenges. Use APIs and middleware that facilitate seamless integration with existing systems. Additionally, involve IT teams early in the process to ensure compatibility and minimize disruptions. Efficient integration will be crucial as nearly 33% of IT leaders aim to make generative AI their top priority.
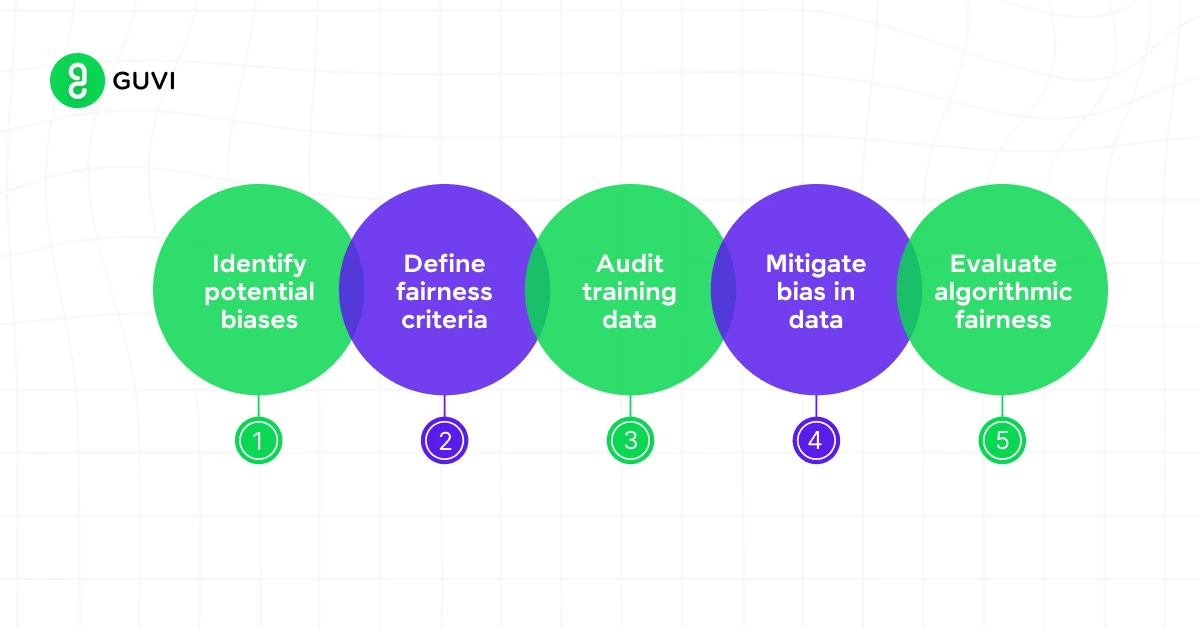
10. Governance and Regulatory Compliance
Challenge
Governance is a major obstacle, with over 80% of organizations struggling to establish effective AI governance frameworks, according to a BCG report.
Possible Solution
Develop a comprehensive governance framework that includes clear policies, procedures, and accountability mechanisms for AI use. Stay informed about regulatory developments and ensure compliance with relevant laws and standards. Regularly review and update governance practices to adapt to new challenges, ensuring that AI applications remain ethical and compliant.
Challenge | Possible Solution |
Data Privacy and Security | Implement anonymization and encryption; adhere to data governance policies. |
Bias in AI Models | Use diverse datasets; conduct regular bias audits and involve interdisciplinary teams. |
Ethical Concerns | Develop and enforce ethical guidelines; label AI-generated content and detect deepfakes. |
Lack of Strategic Roadmap | Create a clear AI strategy with measurable goals; involve senior leadership in planning. |
Scalability Issues | Utilize cloud-based platforms; implement modular AI frameworks for incremental scaling. |
Lack of Skilled Workforce | Invest in employee training; collaborate with educational institutions for AI programs. |
High Computational Costs | Optimize models for efficiency; use AIaaS solutions and energy-efficient hardware. |
Quality Control | Implement validation processes; use human-in-the-loop systems and update models regularly. |
Integration with Existing Systems | Start with pilot projects; use APIs and middleware for seamless integration. |
Governance and Regulatory Compliance | Develop governance frameworks; stay updated on regulations and ensure compliance. |
Generative AI presents both exciting possibilities and formidable challenges. Addressing these challenges through strategic planning, ethical considerations, and continuous innovation will be key to harnessing the full potential of this transformative technology. As companies prioritize generative AI, with 43% already testing tools and products, it’s crucial to remain vigilant in navigating these challenges to drive productivity and creativity across industries.
Looking Ahead: The Future of Generative AI
The future of generative AI promises to be transformative, with advancements in multimodal generation, personalized AI companions, and AI-human hybrid creativity. We can anticipate AI systems capable of seamlessly generating and understanding content across text, image, audio, and even tactile or olfactory information, leading to new immersive experiences.
Additionally, highly personalized AI companions may offer emotional support and assist with various tasks, while AI-human collaborations could blur the lines between human and AI-generated content, fostering new forms of creativity.
The future might also see the emergence of ethical AI agents with sophisticated moral reasoning and quantum-enhanced generation capabilities, potentially unlocking unprecedented generative potential. As these developments unfold, it’s crucial to engage in dialogue about their societal implications and develop adaptive governance frameworks.
If you’re ready to learn AI and ML, the Artificial Intelligence and Machine Learning Courses by GUVI is your perfect gateway. Gain hands-on experience, learn from industry experts, and get endless opportunities in one of the most exciting fields today. Enroll now and start your journey toward becoming an AI and ML expert!

Conclusion
Generative AI is one of the most powerful and transformative technologies of our time. Its potential to augment human intelligence, accelerate innovation, and open possibilities is immense. At the same time, it presents profound challenges that cut to the heart of how we relate to information, creativity, and even reality itself.
Ultimately, the future of generative AI – whether it leads to a flourishing of human creativity and progress or to more dystopian outcomes – will depend on the choices we make today and in the coming years. By approaching these technologies with a combination of excitement, caution, and ethical consideration, we can work to steer them toward outcomes that benefit humanity as a whole.
One thing is clear – the possibilities are limited only by our imagination, and the challenges by our wisdom in shepherding these powerful tools. The journey ahead promises to be one of the most consequential and exciting in human history.
FAQs
What ethical concerns are associated with Generative AI?
Generative AI raises ethical issues like copyright infringement, as models use existing works to generate new content, and the creation of deepfakes and misinformation. Bias in training data is another concern, potentially perpetuating or amplifying societal biases.
How does Generative AI impact productivity and creativity?
Generative AI enhances productivity by automating routine tasks, freeing professionals to focus on higher-level work. It also fuels creativity by generating novel ideas and designs, leading to innovative breakthroughs across industries.
What challenges exist in ensuring reliable and high-quality Generative AI outputs?
Challenges include managing inaccuracies (hallucinations), maintaining consistency in longer content, and ensuring data quality. Ongoing model refinement and robust validation are essential for reliable, high-quality outputs.
How can Generative AI be used responsibly?
Responsible use of Generative AI involves setting clear guidelines, monitoring outputs for bias, and ensuring transparency in how the AI is trained and used, along with strong governance to prevent misuse.
What are the future trends in Generative AI?
Future trends include multimodal generation across text, image, and audio, personalized AI companions, AI-human hybrid creativity, and AI’s role in scientific discovery, driving innovation in various fields.
This course was very useful for information to end really good day a AI course was very guvi process