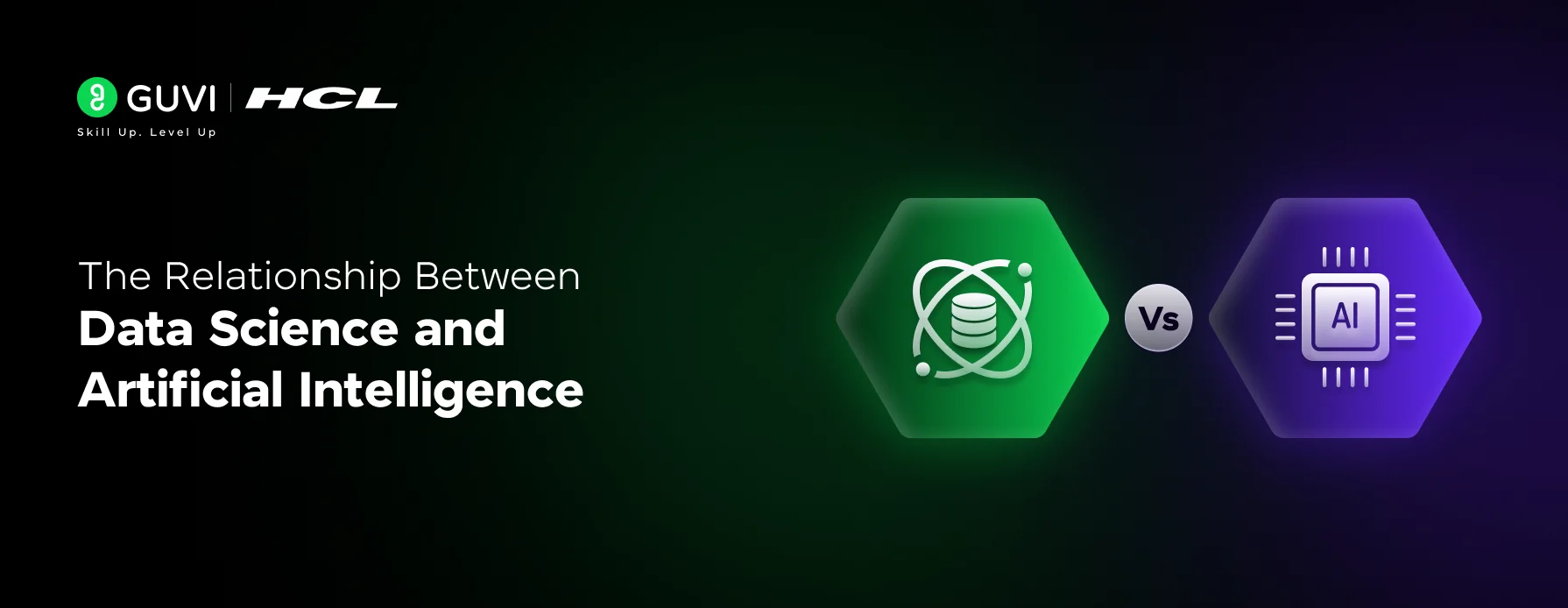
The Relationship Between Data Science and Artificial Intelligence
Apr 01, 2025 8 Min Read 4776 Views
(Last Updated)
In today’s data-driven world, extracting meaningful insights from vast datasets is essential. This is where Data Science and Artificial Intelligence (AI) come into play. Data Science focuses on collecting, cleaning, analyzing, and visualizing data, providing the foundation for understanding complex information. AI, on the other hand, uses these insights to build intelligent systems that can learn, reason, and make decisions.
Together, Data Science and AI drive innovations across various sectors, from healthcare to finance. Data Science offers the necessary tools and analytical capabilities, while AI enhances these efforts with predictive analytics and automation. This blog will explore their relationship, highlighting key concepts, tools, challenges, and future trends. Let’s begin!
Table of contents
- What is Data Science?
- What is Artificial Intelligence?
- The Relationship of Data Science and Artificial Intelligence: Shaping the Future of Technology
- The Intersection of Data Science and AI
- Challenges and Ethical Considerations
- Future Trends: The Evolving Landscape of Data Science and AI
- Conclusion
- FAQs
- What is the difference between Data Science and Artificial Intelligence?
- How do Data Science and AI work together?
- What are some real-world applications of Data Science and AI?
What is Data Science?
Data science is an interdisciplinary field that uses scientific methods, processes, algorithms, and systems to extract knowledge and insights from structured and unstructured data. It combines aspects of:
- Statistics
- Mathematics
- Computer science
- Information science
- Domain expertise
Key components of data science include:
- Data collection and cleaning
- Exploratory data analysis
- Machine learning and predictive modeling
- Data visualization
- Big data processing
Data scientists work on solving complex problems and making data-driven decisions in various industries, from business and finance to healthcare and technology.
Before we move into the next section, ensure you have a good grip on data science essentials like Python, MongoDB, Pandas, NumPy, Tableau & PowerBI Data Methods. If you are looking for a detailed course on Data Science, you can join GUVI’s Data Science Course with Placement Assistance. You’ll also learn about the trending tools and technologies and work on some real-time projects.
Additionally, if you want to explore Python through a self-paced course, try GUVI’s Python course.
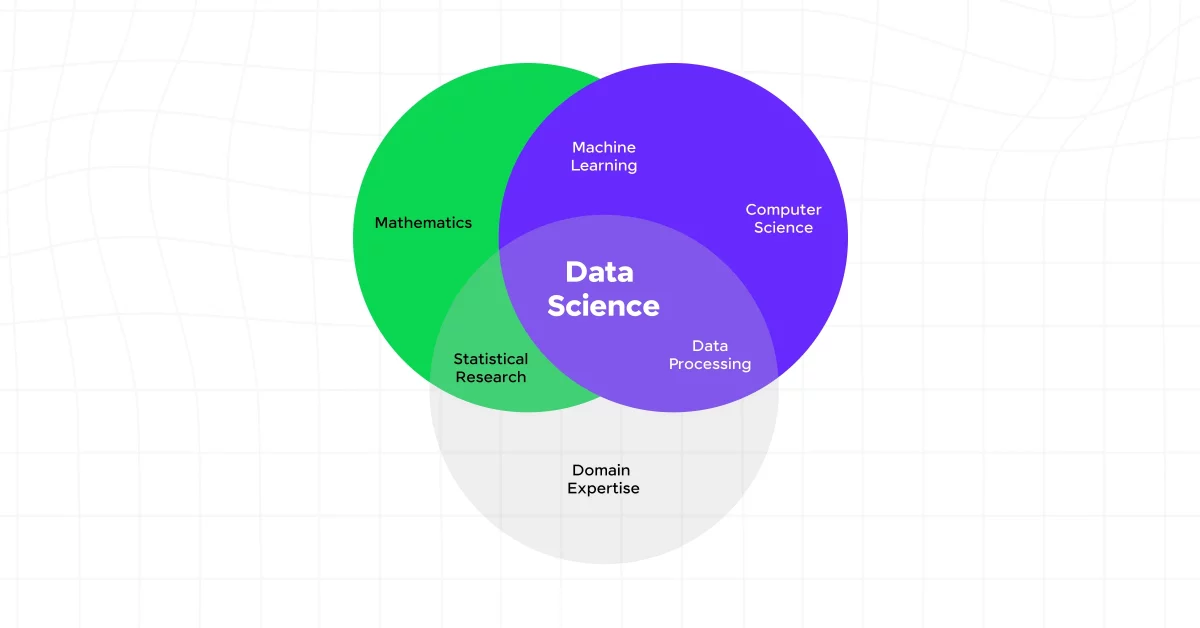
As we explore data science, it’s essential to understand its close companion: Artificial Intelligence (AI). Let’s learn what AI is all about.
What is Artificial Intelligence?
Artificial Intelligence (AI) refers to the simulation of human intelligence in machines that are programmed to think and learn like humans. It encompasses the development of computer systems capable of performing tasks that typically require human intelligence. Key aspects of AI include:
- Machine Learning: Algorithms that improve through experience
- Natural Language Processing: Enabling computers to understand and generate human language
- Computer Vision: Allowing machines to interpret and understand visual information
- Robotics: Creating machines that can interact with the physical world
AI can be categorized into:
- Narrow AI: Designed for specific tasks (e.g., voice assistants, image recognition)
- General AI: Hypothetical AI with human-like cognitive abilities across various domains
AI is applied in numerous fields, including:
- Healthcare (diagnosis, drug discovery)
- Finance (fraud detection, algorithmic trading)
- Transportation (autonomous vehicles)
- Customer service (chatbots)
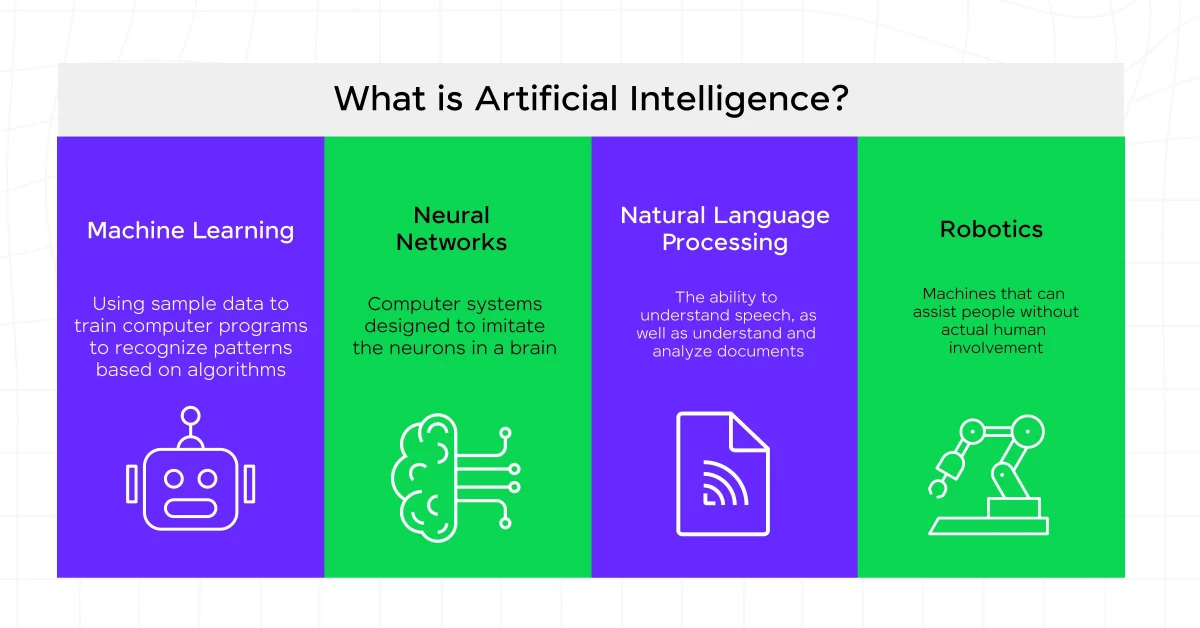
Now that we’ve covered the basics of Artificial Intelligence, let’s explore how the synergy of Data Science and AI is shaping the future of technology.
If you want to learn data science, explore how long it takes to master this in-demand skill and start your data science journey today! Read the full blog now and start learning!
The Relationship of Data Science and Artificial Intelligence: Shaping the Future of Technology
Let’s explore how Data Science and AI are not just a technological advancement, but a shift in how we approach problem-solving, decision-making, and our understanding of the world around us.
The Intersection of Data Science and AI
At first glance, Data Science and Artificial Intelligence might seem like two sides of the same coin. While they do share common ground and often work in tandem, understanding their unique characteristics and how they intersect is important to appreciating their combined power.
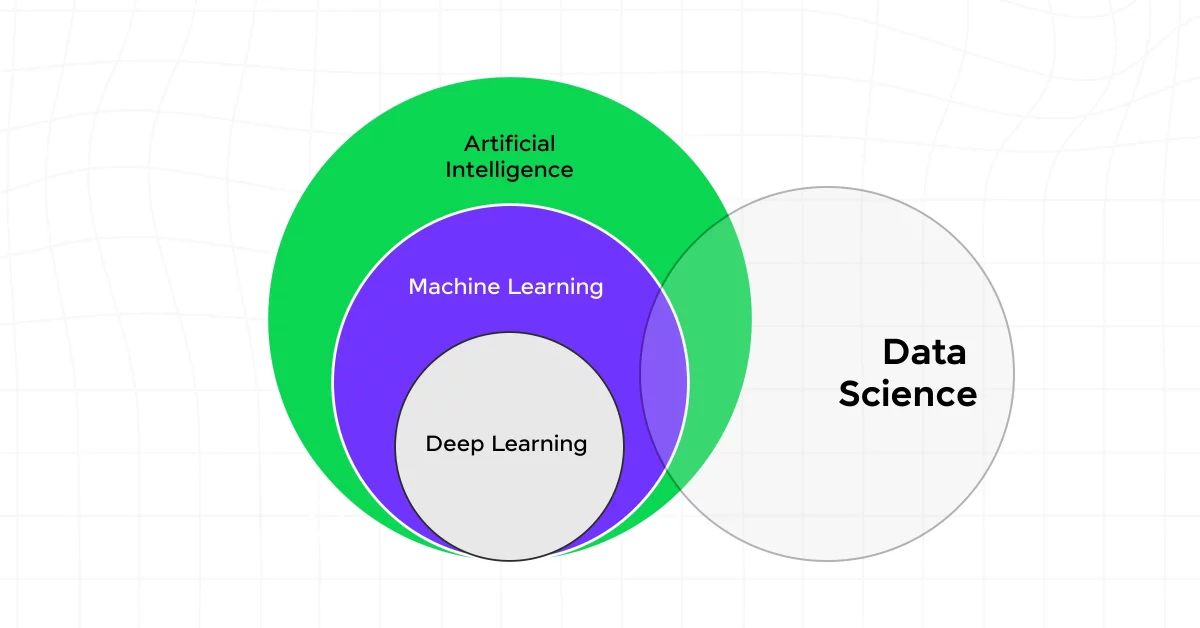
Data Science: The Foundation
Data Science is fundamentally about extracting insights and knowledge from data. It encompasses a wide range of techniques and methodologies, including statistical analysis, data mining, predictive modeling, and data visualization. Data scientists are often described as part statistician, part computer scientist, and part business strategist. They work to collect, process, and analyze vast amounts of structured and unstructured data to uncover patterns, trends, and insights that can inform decision-making and strategy.
If you’ve ever dreamed of becoming a data scientist, now is your chance! Follow this complete guide on data science and transform your career in just 3 months.
Artificial Intelligence: The Frontier
Artificial Intelligence, on the other hand, focuses on creating systems that can perform tasks that typically require human intelligence. This includes learning, problem-solving, perception, and language understanding. AI systems aim to mimic cognitive functions, make decisions, and improve their performance over time through experience.
The Relationship
The intersection of Data Science and AI is where the magic happens. Here’s how they complement each other:
- Data as Fuel for AI: AI systems, particularly machine learning models, require vast amounts of high-quality data to train and improve. Data Science provides the methodologies to collect, clean, and prepare this data, ensuring that AI systems have the robust foundation they need to learn and make accurate predictions or decisions.
- AI Enhancing Data Analysis: While traditional Data Science syllabus can uncover insights from data, AI takes this a step further. Machine learning algorithms can identify complex patterns and relationships in data that might be invisible to human analysts or traditional statistical methods. This allows for more sophisticated and accurate predictions and insights.
- Automated Feature Engineering: In Data Science, feature engineering – the process of selecting and transforming variables for a machine learning model – is often a time-consuming, manual process. AI techniques like automated machine learning (AutoML) can automate this process, identifying the most relevant features and even creating new ones, thus accelerating the Data Science workflow.
- Handling Unstructured Data: A significant challenge in Data Science is dealing with unstructured data like text, images, and video. AI techniques, particularly in natural language processing and computer vision, excel at extracting meaningful information from these unstructured sources, expanding the scope of what Data Science can analyze.
- Continuous Learning and Adaptation: While traditional Data Science models might be static, AI systems can continuously learn and adapt as new data becomes available. This creates a dynamic feedback loop where the insights generated by Data Science inform and improve AI models, which in turn enhance the Data Science capabilities.
- Explainable AI and Data Interpretation: As AI models become more complex, there’s a growing need for explainable AI – systems that can provide clear reasoning for their decisions. Data Science techniques play an important role in interpreting and visualizing the outputs of AI models, making them more transparent and trustworthy.
- Predictive to Prescriptive Analytics: Data Science traditionally focuses on descriptive and predictive analytics – understanding what happened and what might happen. AI takes this further into prescriptive analytics, not just predicting outcomes but recommending actions to achieve desired results.
The synergy between Data Science and AI is not just theoretical; it’s driving innovations across industries:
- Healthcare: Combining data analysis with AI is revolutionizing diagnostics, drug discovery, and personalized medicine. For example, AI models trained on vast datasets of medical images can detect diseases like cancer with accuracy rivaling or surpassing human experts.
- Finance: In the financial sector, this intersection is powering advanced fraud detection systems, algorithmic trading, and personalized financial advice. AI models can analyze market data in real-time, making split-second trading decisions based on complex patterns.
- Marketing: AI-powered data analysis enables hyper-personalized marketing campaigns. By analyzing consumer behavior data, AI systems can predict individual preferences and tailor marketing messages accordingly.
- Smart Cities: Urban planners are using the combination of Data Science and AI to optimize traffic flow, reduce energy consumption, and improve public services. AI models analyze data from various sensors and sources to make real-time decisions and predictions.
- Environmental Conservation: From predicting wildlife movements to monitoring deforestation, the intersection of Data Science and AI is providing powerful tools for environmental protection. Satellite imagery combined with AI analysis can track changes in ecosystems over time with unprecedented accuracy.
As we continue to explore the relationship between Data Science and AI, it becomes clear that their intersection is a launchpad for innovation. This synergy is pushing the boundaries of what’s possible, enabling us to tackle complex problems and make more informed decisions across all sectors of society.
However, as with any transformative technology, the integration of Data Science and AI also presents significant challenges and ethical considerations. In the next section, we’ll explore these important aspects, examining the hurdles that must be overcome and the ethical questions that must be addressed as we continue to advance these technologies.
Challenges and Ethical Considerations
While the fusion of Data Science and AI offers tremendous potential, it also presents significant challenges and raises important ethical questions. As we continue to push the boundaries of what’s possible with these technologies, it’s important to address these issues head-on.
Technical Challenges
- Data Quality and Quantity: The effectiveness of AI models is heavily dependent on the quality and quantity of data used to train them. Ensuring data is accurate, comprehensive, and representative is an ongoing challenge.
- Issue: Biased or incomplete datasets can lead to skewed results and reinforcement of existing prejudices.
- Potential Solution: Implementing rigorous data collection and cleaning processes, and using techniques like data augmentation to address imbalances.
- Model Interpretability: As AI models become more complex, understanding how they arrive at their decisions becomes increasingly difficult.
- Issue: This “black box” problem can lead to a lack of trust and difficulty in identifying and correcting errors.
- Potential Solution: Developing “explainable AI” techniques that provide insight into the decision-making process of AI models.
- Scalability and Computational Resources: Training and running sophisticated AI models requires significant computational power.
- Issue: This can be a barrier to entry for smaller organizations and can have substantial environmental impacts due to energy consumption.
- Potential Solution: Developing more efficient algorithms and exploring alternative computing architectures like quantum computing.
- Integration of AI Systems: Implementing AI solutions in existing business processes and legacy systems can be challenging.
- Issue: Resistance to change and technical incompatibilities can hinder adoption.
- Potential Solution: Gradual implementation strategies and development of middleware solutions to bridge old and new systems.
Ethical Considerations
- Privacy Concerns: The vast amounts of data required for AI and Data Science raise significant privacy issues.
- Issue: Collection and use of personal data without adequate consent or protection.
- Consideration: Implementing robust data protection measures and adhering to regulations like GDPR. Developing privacy-preserving AI techniques such as federated learning.
- Bias and Fairness: AI systems can perpetuate and amplify existing societal biases.
- Issue: Discriminatory outcomes in areas like hiring, lending, and criminal justice.
- Consideration: Regular audits of AI systems for bias, diverse representation in AI development teams, and implementation of fairness constraints in algorithms.
- Job Displacement: As AI systems become more capable, there’s concern about widespread job losses.
- Issue: Potential economic disruption and social unrest.
- Consideration: Focusing on AI as an augmentation to human capabilities rather than a replacement. Investing in retraining and education programs to prepare the workforce for AI-driven changes.
- Accountability and Liability: As AI systems make more critical decisions, questions of accountability arise.
- Issue: Determining responsibility when AI systems make errors or cause harm.
- Consideration: Developing clear regulatory frameworks for AI accountability. Ensuring human oversight in critical decision-making processes.
- Autonomous Weapons and Security: The potential use of AI in warfare raises serious ethical concerns.
- Issue: Development of lethal autonomous weapons and potential for AI-powered cyber attacks.
- Consideration: International agreements on the use of AI in military applications. Robust cybersecurity measures to protect against AI-powered threats.
- Transparency and Informed Consent: There’s often a lack of transparency about how personal data is used in AI systems.
- Issue: Individuals may not fully understand how their data is being used or the implications of AI-driven decisions affecting them.
- Consideration: Implementing clear disclosure policies and obtaining meaningful informed consent for data use.
- Environmental Impact: The energy consumption of large-scale AI systems is a growing concern.
- Issue: Significant carbon footprint of data centers and AI training processes.
- Consideration: Investing in energy-efficient computing technologies and exploring ways to optimize AI algorithms for lower energy consumption.
- Digital Divide: The benefits of AI and Data Science may not be equally distributed.
- Issue: Widening gap between those who have access to AI technologies and those who don’t.
- Consideration: Efforts to make AI technologies more accessible and to ensure equitable distribution of their benefits.
Addressing these Challenges
To tackle these challenges and ethical considerations, a multi-faceted approach is necessary:
- Regulatory Frameworks: Developing comprehensive, flexible regulations that can keep pace with technological advancements.
- Ethical Guidelines: Establishing industry-wide ethical standards for the development and deployment of AI systems.
- Education and Awareness: Improving public understanding of AI and Data Science, their capabilities, and their limitations.
- Interdisciplinary Collaboration: Encouraging cooperation between technologists, ethicists, policymakers, and domain experts to address these complex issues.
- Ongoing Research: Continuing to invest in research on ethical AI, explainable AI, and privacy-preserving technologies.
- Diverse Representation: Ensuring diverse perspectives are included in the development and governance of AI systems.
As we navigate these challenges, it’s important to remember that the goal is not to impede progress, but to ensure that the future of Data Science and AI aligns with our ethical values and societal needs. By addressing these issues proactively, we can use the full potential of these technologies while mitigating their risks.
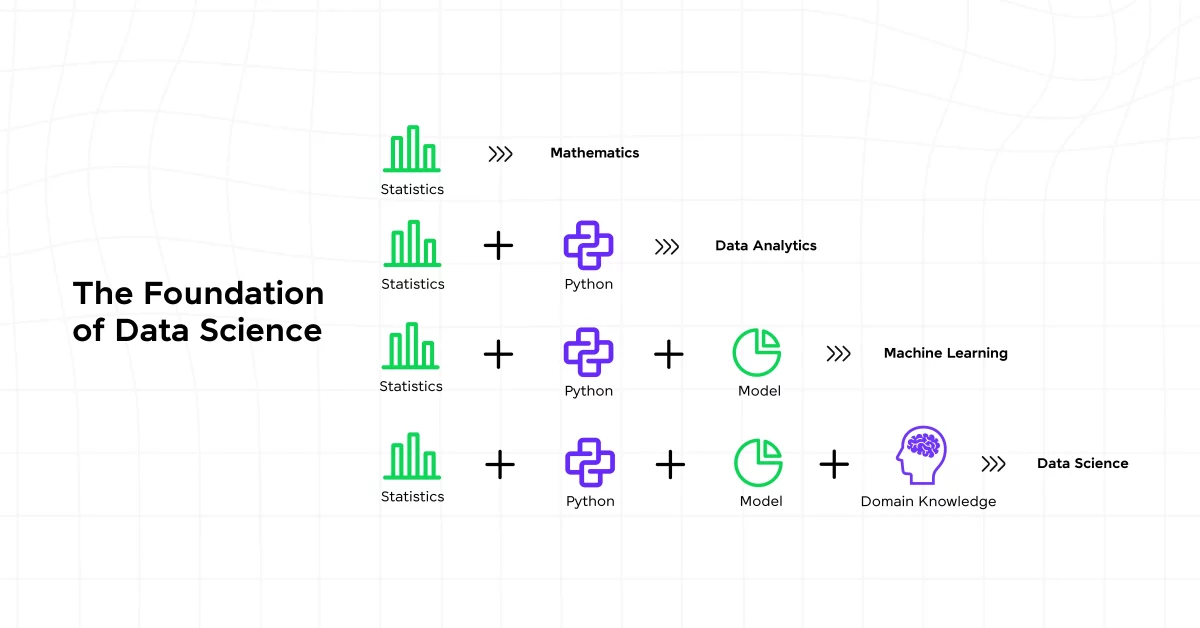
In the final section, we’ll look ahead to the future, exploring emerging trends and potential developments in the intersection of Data Science and AI.
Future Trends: The Evolving Landscape of Data Science and AI
As we look to the horizon, the intersection of Data Science and AI continues to evolve rapidly, promising exciting developments and transformative possibilities. Here are some key trends that are likely to shape the future of these intertwined fields:
- AutoML and AI-Assisted Data Science: Automated Machine Learning (AutoML) tools are becoming increasingly sophisticated, making AI more accessible to non-experts.
- Future Impact: We can expect AI to take on more of the heavy lifting in data preprocessing, feature engineering, and model selection. This democratization of AI will allow a broader range of professionals to use its power, potentially leading to innovations across various domains.
- Edge AI and Distributed Learning: As IoT devices increase, there’s a growing trend towards processing data at the edge rather than in centralized cloud servers.
- Future Impact: This will enable real-time AI applications with reduced latency and improved privacy. Federated learning, where models are trained across multiple decentralized devices, will become more prevalent.
- AI-Augmented Analytics: The integration of AI into traditional business intelligence tools is giving rise to more intuitive, conversational interfaces for data analysis.
- Future Impact: This will empower non-technical users to perform complex data analysis, potentially leading to more data-driven decision-making across all levels of organizations.
- Quantum Computing in AI and Data Science: While still in its early stages, quantum computing holds immense potential for revolutionizing AI and Data Science.
- Future Impact: Quantum algorithms could dramatically speed up machine learning processes and enable the tackling of currently intractable computational problems.
- Ethical AI and Responsible Data Science: As discussed in the previous section, addressing ethical concerns will be important for the sustainable development of AI and Data Science.
- Future Impact: We can expect to see the emergence of new roles like “AI Ethicist” and the development of more sophisticated tools for detecting and mitigating bias in AI systems.
If you’re eager to transform your career, explore the roles and responsibilities of a data scientist and take the first step towards a rewarding career.
- AI in Scientific Discovery: AI is increasingly being used to accelerate scientific research across various fields.
- Future Impact: We may see AI-driven breakthroughs in areas like drug discovery, materials science, and fundamental physics, potentially revolutionizing the scientific process itself.
- Reinforcement Learning and Autonomous Systems: Advancements in reinforcement learning are paving the way for more sophisticated autonomous systems.
- Future Impact: This could lead to significant developments in robotics, autonomous vehicles, and AI agents capable of complex decision-making in dynamic environments.
- Natural Language Processing and Multimodal AI: NLP models are becoming increasingly sophisticated, and there’s a trend towards multimodal AI that can process and generate various types of data (text, image, audio, video).
- Future Impact: This could lead to more natural human-AI interactions and AI systems capable of understanding and generating complex, multi-format content.

Kickstart your Data Science journey by enrolling in GUVI’s Data Science Course where you will master technologies like MongoDB, Tableau, PowerBI, Pandas, etc., and build interesting real-life projects.
Alternatively, if you would like to explore Python through a Self-paced course, try GUVI’s Python certification course.
Conclusion
The fusion of Data Science and AI is not just shaping our technological landscape; it’s redefining how we interact with information, make decisions, and solve complex problems. As we’ve explored in this blog post, from healthcare to climate science, from ethical considerations to future trends, the impact of this synergy is profound and far-reaching.
However, as we await these exciting possibilities, we must remain mindful of the challenges and ethical considerations. The future of Data Science and AI should not just be about what we can do, but what we should do. By fostering responsible innovation, prioritizing ethical considerations, and ensuring equitable access to these technologies, we can use their full potential to create a better, more informed, and more sustainable world.
As we move forward, it’s clear that the intersection of Data Science and AI will continue to drive innovation and progress. Staying informed about these developments will be important in navigating the data-driven, AI-enhanced future.
FAQs
Data Science is a field focused on extracting insights from data using techniques like statistics, data analysis, and machine learning. It involves data collection, cleaning, analysis, and visualization. Artificial Intelligence (AI) involves creating systems that can perform tasks requiring human intelligence, such as learning and problem-solving.
Data Science and AI are interdependent. Data Science offers the tools and methods to collect and analyze data, which AI models use to learn and make decisions. AI enhances Data Science by automating data analysis, making predictions, and finding hidden patterns. Together, they create intelligent systems capable of advanced problem-solving and decision-making, driving innovation across various fields.
Data Science and AI are used in many industries. In healthcare, they power diagnostic tools and personalized treatments. In finance, they help with fraud detection and algorithmic trading. Retail and e-commerce use them for customer behavior predictions and inventory management. In manufacturing, they aid in predictive maintenance and quality control.
Did you enjoy this article?