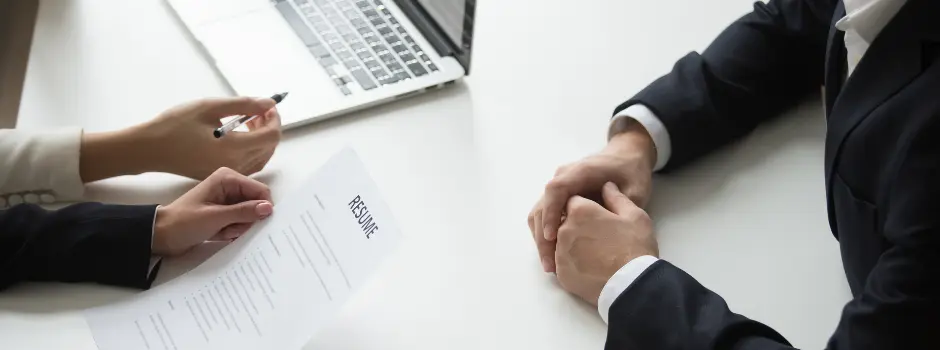
Guide for Data Scientist Resume and Portfolio Creation
Mar 05, 2025 6 Min Read 2316 Views
(Last Updated)
The U.S. Bureau of Labor Statistics (BLS) anticipates a 36% growth rate for data scientist positions from 2023 to 2033, which is much faster than the average for all occupations.
What does this mean? This simply means that the demand for data scientists is increasing each day, in each sector. Data science applications are expanding into various sectors, including healthcare, finance, e-commerce, and more. For instance, 56% of healthcare centers have adopted predictive analytics, highlighting its importance in improving patient outcomes and operational efficiency.
A well-crafted portfolio and a resume tell the employer or the potential client more than just your achievements and accomplishments. It tells them in a straight, solid way that being a data scientist is more than a day job for you and how passionate you are about this field.
If you are struggling with where to start your career even with a great knowledge of Data Science on your backpack, this post helps you with that. This blog post helps you take the first step – the scary, intimidating one with ease. Your first task to work on when you are looking for a data scientist job is to fix your resume and portfolio. Once that is ready, we must tell you that everything will eventually fall into place.
This blog is tailored for both aspiring and seasoned data scientists looking to create standout resumes and portfolios that secure interviews. Expect practical steps, examples, and tools to build a professional and compelling showcase of your skills, setting you apart in the competitive field of data science.
Table of contents
- Why Your Resume and Portfolio Are Crucial in Data Science
- Why Are Both Important?
- Crafting a Data Scientist Resume That Gets Noticed
- Formatting tips to ensure readability and ATS compatibility
- A few things to keep in mind
- Building a Data Science Portfolio That Stands Out
- What to Include in a Data Science Portfolio
- Tools and Platforms to Build Your Data Science Portfolio
- Addressing the 'No Experience' Challenge
- Wrapping it all together
- FAQ
Why Your Resume and Portfolio Are Crucial in Data Science
Data science is a lucrative career with often amazing pay. Your resume and portfolio are your entry tickets to this field, showcasing your skills, projects, and real-world applications. A strong resume highlights your technical expertise, while a well-crafted portfolio demonstrates your ability to solve problems using data, setting you apart in this competitive domain.
While some may debate which is more crucial between a resume and a portfolio, let’s tell you the fact out loud that both of them are highly crucial for you to land your dream job or your dream client.
Your portfolio, showcasing the projects you have worked on, your technical skills, and the tools you are proficient in, complements your resume, which is brief, concise, and to the point.
For someone switching jobs, drafting a resume may be a cakewalk, as they likely understand what works and what doesn’t. However, for fresh graduates unsure where to start with their resume and portfolio, this guide will serve as a valuable resource to refer back to and iterate upon – making it worth your time.
Why Are Both Important?
- A resume provides a snapshot of your qualifications and experiences, while a portfolio offers an in-depth view of how you apply those skills in real-world scenarios. Together, they create a comprehensive picture of your capabilities.
- A well-crafted portfolio serves as tangible proof of your skills and accomplishments. While resumes list qualifications, portfolios showcase actual work and results, building trust with potential employers.
- By using both a resume and portfolio strategically, you can tailor your applications to specific roles. The resume can highlight relevant experiences succinctly, while the portfolio can showcase detailed projects that align with the job requirements.
In conclusion, both a resume and a portfolio are crucial in the data science field. They complement each other by providing a full spectrum of your professional identity, one that combines formal qualifications with practical applications of your skills.
Crafting a Data Scientist Resume That Gets Noticed
When employers review a data scientist’s resume, they evaluate it with critical questions in mind to decide if you’re a fit for the role. Here are the questions they often ask:
- Is the resume easy to scan?
Employers typically spend only 6–10 seconds on a resume initially. If it’s cluttered or hard to navigate, it’s likely to get overlooked. - Is the resume visually appealing?
A clean, professional design with consistent fonts and spacing enhances readability and gives a positive impression. - Does it highlight measurable outcomes?
Employers want evidence of your impact—metrics like “improved model accuracy by 25%” or “reduced costs by 10%” are far more compelling than vague descriptions. - Are the skills relevant to the role?
They scan for tools, languages, and technologies mentioned in the job description. Unrelated or overly generic skills dilute the resume’s effectiveness. - Does it demonstrate a mix of technical and business skills?
Data scientists don’t just crunch numbers; they translate insights into actionable business strategies. Resumes should reflect this dual capability. - Are the projects unique and practical?
Employers appreciate innovative, real-world examples over commonly seen academic projects. This shows creativity and real-world problem-solving. - Is the experience tailored to the industry?
Highlighting domain-specific experience in sectors like finance, healthcare, or retail can make your resume stand out.
Answering these questions positively through your resume will set you apart as a candidate who not only has the technical skills but also the strategic and practical expertise needed for the role.
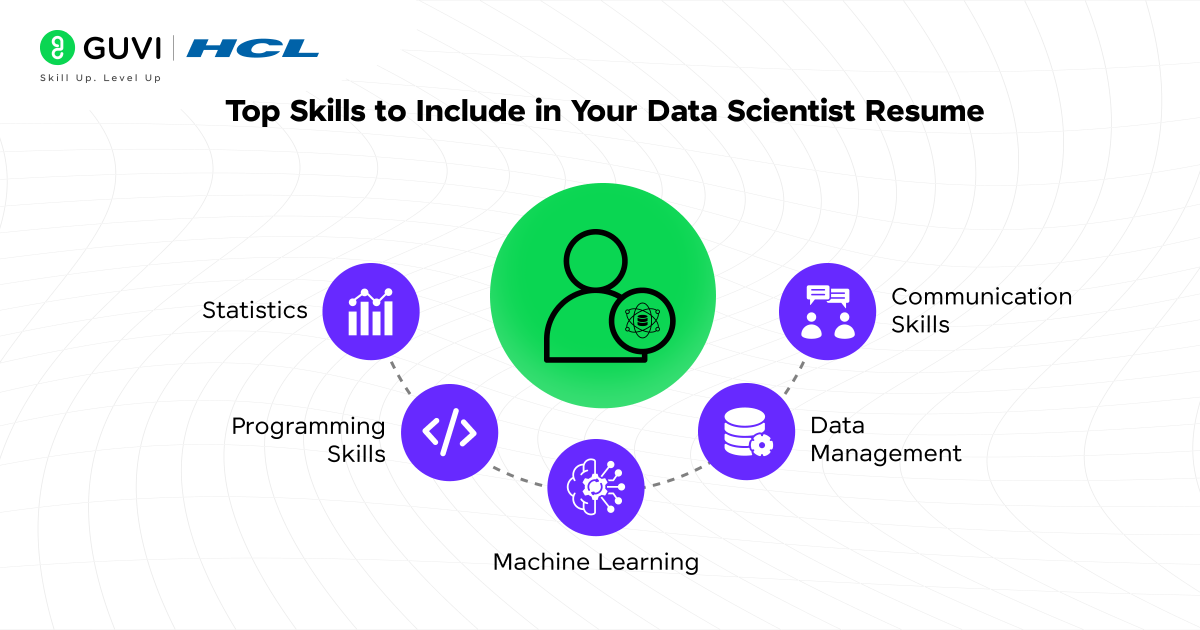
Formatting tips to ensure readability and ATS compatibility
To give a quick overview of what ATS is – ATS, or the Applicant Tracking System, is software that helps organizations manage a high volume of applicants by sorting, filtering, and sometimes scoring resumes based on predefined criteria. It plays a critical role in the initial stages of recruitment.
As much as this tool can be a savior for large organizations, it can sometimes be a threat to applicants who are unaware of the intricacies of how ATS works. For example – ATS can’t analyze resumes with a lot of graphics, tables, and multiple columns. And ATS primarily screens candidates based on the keywords that match the job description. Missing these two factors while crafting your resume can cost you great opportunities.
Here are a few key things to keep in mind while crafting your resume:
1. Optimize the keywords
- Tailor your resume to the job description by including specific skills, tools, and experiences mentioned in the posting.
- Use exact phrasing wherever possible, e.g., “machine learning” instead of “AI techniques.”
- Avoid keyword stuffing; ensure terms are used naturally within your accomplishments or skills list.
2. Stick to simple formatting
Use a single-column layout with standard headings like “Education,” “Experience,” and “Skills.” Avoid images, tables, columns, or charts, as these can confuse ATS systems.
3. Save incompatible file formats
In most cases, the preferred file format would be .pdf format or .docx. Refer to the job application instructions for the preference mentioned and proceed accordingly.
4. Select Appropriate Fonts and Sizes
Use standard fonts such as Arial, Calibri, or Times New Roman in sizes 10-12 pt for body text and 14-16 pt for headings. This ensures readability for ATS and human reviewers while maintaining a professional appearance.
A few things to keep in mind
- Avoid overloading your resume with technical jargon. Use plain, concise language to describe your expertise, ensuring that both technical and non-technical recruiters can understand your accomplishments.
- Dedicate a section to showcase key projects that demonstrate your data science skills. Include a brief description of each project, the methodologies used, and the outcomes achieved. Use bullet points for easy readability.
- Along with listing programming languages, frameworks, and tools (e.g., Python, TensorFlow, SQL), highlight soft skills like data storytelling, stakeholder communication, and business strategy alignment to demonstrate your end-to-end capabilities.
Building a Data Science Portfolio That Stands Out
A collection of your best work and projects often speaks louder about your capabilities than a brief resume. While a resume and portfolio are non-negotiable, a portfolio can serve as your golden ticket – be it with an employer or a potential client.
A resume may help you get the first “Yes” and secure an initial interview or call, but in the field of data science, where problem-solving and technical expertise are highly important. And a solid work proof can spin wonders.
How portfolios give recruiters a clearer picture of your skills
Decision-making requires solid proof and evidence and unlike resumes, which often focus on qualifications and job titles, portfolios allow you to present detailed case studies, visualizations, and project outcomes.
This comprehensive view helps recruiters assess your technical skills, creativity, and problem-solving capabilities more effectively. A portfolio demonstrates how you apply data science in practical, industry-specific scenarios. Data science is a multidisciplinary field and a portfolio can reflect your adaptability by featuring projects in various domains, languages, or techniques, offering a comprehensive picture of your capabilities.
A portfolio can also serve as a great way to demonstrate how you have evolved in the industry as a data scientist. This projection of continuous growth in your portfolio can be a great convincing factor for your employer.
What to Include in a Data Science Portfolio
Here we go with the cliched and outdated statement – “Less is more” when it comes to either your portfolio or your resume. One thing to avoid primarily is cluttering your portfolio with unnecessary information that is irrelevant to the job description – such as unrelated hobbies, old coursework, or projects that don’t showcase your relevant skills. Instead, focus on showcasing high-impact projects that align with the skills and technologies required for the data science roles you’re applying for.
For example, imagine you’re applying for a data science position at a company that heavily uses Python, machine learning, and large-scale data analysis. Instead of including a project about basic data cleaning (which might be too simplistic for the role), you could feature a machine learning model you built to predict customer churn using a large dataset.
Here are a few things you must include in your portfolio:
- A short introduction about yourself
Introduce who you are and what you specialize in as a data scientist. Keep it concise yet informative, highlighting your technical expertise, core skills, and career goals.
- Your best works and projects
Showcase a selection of your most impactful projects, specifying the type of work involved—such as predictive modeling, data visualization, or machine learning applications. Each project should demonstrate your technical skills and problem-solving abilities, providing clear examples of how you’ve applied data science concepts in real-world scenarios.
- Social proof/ testimonials
Include quotes or references from past clients, mentors, or colleagues that vouch for your skills and work ethic. This builds credibility and shows potential employers or clients that you have a track record of success.
- Contact information
Ensure that it’s easy for potential employers or collaborators to reach you. Include your professional email, and links to your LinkedIn profile, GitHub, or personal website.
Pro tip: Words can’t always justify and express your work, the approach you took, and the problems you have solved so far as a data scientist. So make sure to present your portfolio in a visually pleasing way. Use charts, graphs, and dashboards to effectively present your achievements.
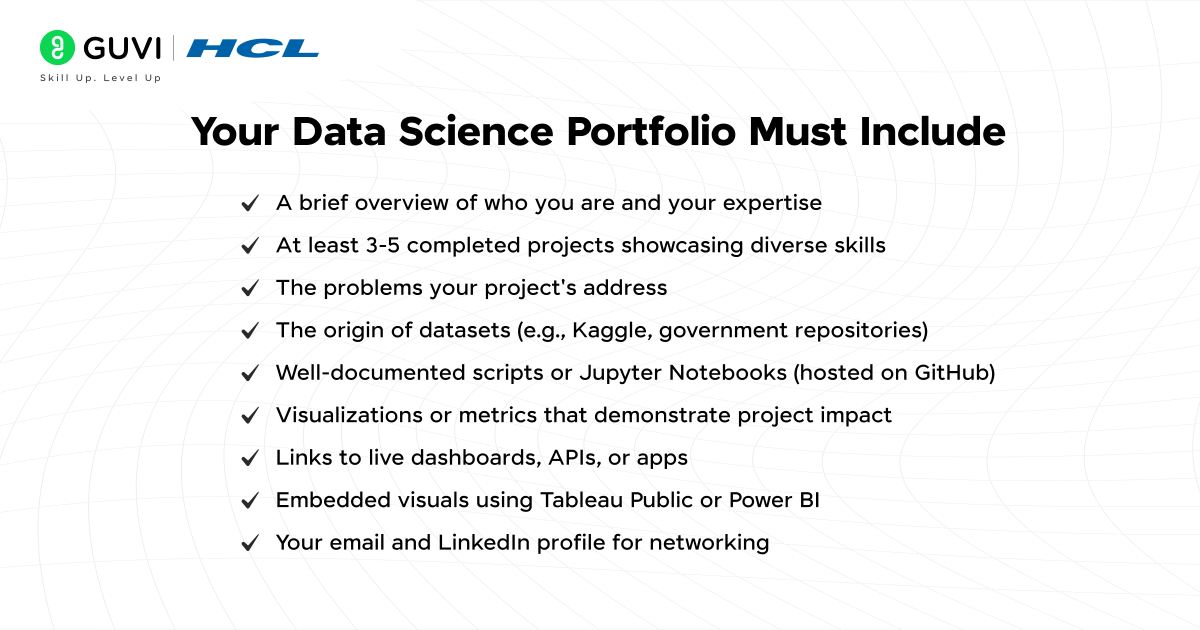
Tools and Platforms to Build Your Data Science Portfolio
Tools such as GitHub, DataCamp, Behance, Tableau Public, and Jupyter Notebooks can be used to create your portfolio as a data scientist.
Addressing the ‘No Experience’ Challenge
As a data scientist, especially when you are just starting, it can be hard to present either your resume or your portfolio without any prior experience. And we have mapped a few ways for you to overcome this challenge.
1. Highlight Your Programming Abilities and Proficiency in Languages
As a data scientist, programming is one of your core strengths. And it is essential to highlight your proficiency in programming languages used in the field such as Python, R, SQL, and sometimes Java or C++.
Use specific language-related keywords like “Python for data analysis,” “R for statistical modeling,” or “SQL for database queries.”
2. Showcase Your Code
Use platforms like GitHub to host your code repositories related to your projects for your portfolio. This not only demonstrates your coding skills but also allows recruiters to see your thought process and problem-solving approach.
3. Highlight Relevant Skillsets and Tools Learned
As a data scientist, the tools and techniques you use are just as important as the programming languages. Mention your proficiency with tools like Pandas, Numpy, Scikit-learn, Matplotlib, and TensorFlow for data analysis, machine learning, and visualization. Also, don’t forget to include version control tools like Git or cloud platforms like AWS or Google Cloud.
Transform Your Passion into a Data Science Career
The demand for skilled data scientists has never been higher, and your resume and portfolio are your golden tickets to landing the opportunities you deserve. But what if you could go beyond just crafting impressive documents?
Our Data Science Course is designed to empower you with the skills, tools, and real-world experience to build projects that will make your portfolio shine. Whether it’s mastering Python, diving into machine learning, or creating dashboards that wow recruiters, this course gives you hands-on learning to transform your career aspirations into reality.
Wrapping it all together
As much as you are keen on developing both a resume and portfolio, you must also be very keen on maintaining consistency and cohesiveness among both of them. Ensure that the projects listed in your resume match those in your portfolio. If you list certain skills on your resume, such as proficiency in Python, data visualization, or machine learning, these skills should also be reflected in the portfolio.
Both your resume and portfolio should be clear and focused. If your resume says you have “experience with large-scale data analysis,” your portfolio should link to projects where you handled datasets of significant size, explaining how you cleaned, analyzed, and derived insights from them.
The field of data science is a constantly evolving one. And if you have to keep up the same pace as it is growing, it is not only essential to stay updated with the tools, and your learning curve but also update your resume and portfolio.
Every three to six months, make sure to review and update both your portfolio and resume.
FAQ
- How to make a data science resume with no experience?
Focus on relevant coursework, certifications, and personal projects that showcase your skills in programming, machine learning, and data analysis. Highlight any internships, volunteer work, or contributions to open-source projects. - How to build a strong portfolio in data science?
Include projects that demonstrate your expertise in real-world data analysis, problem-solving, and technical tools. Use platforms like GitHub or Kaggle to share detailed project reports and codes. - How do I make an impressive portfolio?
Highlight diverse projects (e.g., predictive modeling, data visualization) with clear documentation, visual appeal, and actionable insights. Use storytelling to explain your approach and results. - How to make a data science resume stand out?
Customize your resume for each job by aligning your skills and projects with the job description. Use measurable achievements, and action verbs, and keep it concise yet impactful. - What skills are required for a data scientist?
Core skills include programming (Python, R), data visualization (Tableau, Power BI), statistical analysis, machine learning, and SQL. Problem-solving and communication are equally critical.
Did you enjoy this article?