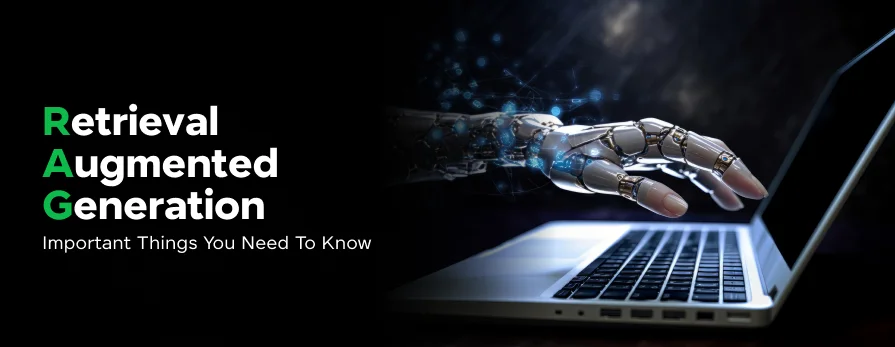
Retrieval Augmented Generation: Important Things You Need To Know About
Dec 21, 2024 6 Min Read 4003 Views
(Last Updated)
What do you think the future of search engines is going to be? As of now, Google is ruling it but with the rise of ChatGPT in late 2022, there’s been a talk on how people will interact with search engines.
If you have used ChatGPT (if not, please do so as you are missing out on a whole lot of things), you must be amused by its prowess in retrieving the information that you requested. Well, there is a whole field of study based on that and it is known as Retrieval Augmented Generation (RAG).
The name’s pretty cool, right? That’s what you are going to learn about in this article, everything there needs to be known about Retrieval Augmented Generation as that is the future of machine learning.
So, without further delay, let’s get started.
Table of contents
- What is Retrieval Augmented Generation?
- The Basics of Retrieval Augmented Generation
- Why is Retrieval Augmented Generation Special?
- RAG’s Broader Impact
- How Retrieval Augmented Generation (RAG) Works?
- Step-by-Step Breakdown of RAG:
- Why is Each Step Important?
- Retrieval Augmented Generation’s Interaction with Data
- Practical Applications of Retrieval Augmented Generation (RAG)
- Enhancing Educational Tools
- Revolutionizing Customer Support
- Transforming Content Creation
- Optimizing Legal and Medical Research
- Improving Accessibility of Information
- Why These Applications Matter?
- Challenges and Considerations of Retrieval Augmented Generation
- Data Quality and Availability
- Integration Complexity
- Computational Costs and Resources
- Privacy and Ethical Concerns
- Scalability Challenges
- Conclusion
- FAQs
- Can RAG be used to generate content in multiple languages?
- What industries could benefit the most from RAG?
- Is there a risk of data bias in RAG systems?
- Can RAG technology be integrated into existing AI systems?
- Can RAG work offline?
What is Retrieval Augmented Generation?
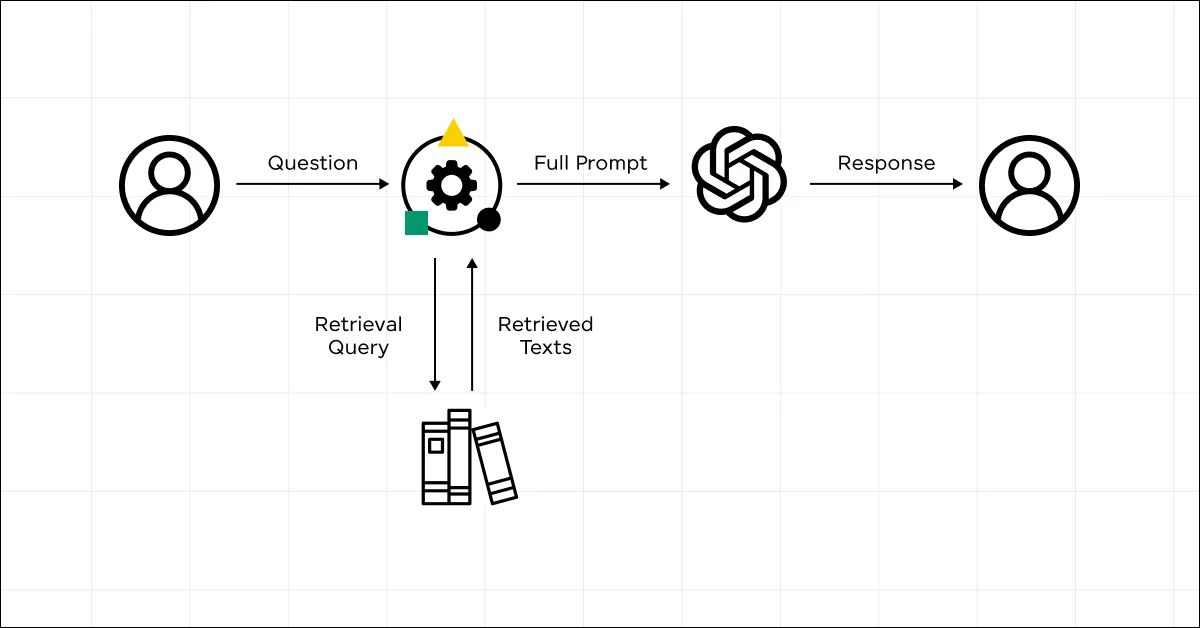
Retrieval Augmented Generation, or RAG, refers to a methodology in machine learning where a system enhances its response generation capability by dynamically retrieving information from a large corpus of data.
It combines the strengths of two distinct AI domains: retrieval-based models and generative models.
The term “Retrieval Augmented Generation” might seem complex at first, but let’s break it down into simpler concepts to better understand how this innovative approach is enhancing the capabilities of artificial intelligence.
Know More: Machine Learning Must-Knows: Reliable Models and Techniques
The Basics of Retrieval Augmented Generation
Imagine you’re writing a research paper; you gather information from various sources and then synthesize it into a perfectly aligned meaningful piece.
The Retrieval Augmented Generation system works similarly but in the digital world. It’s like a smart assistant that can both fetch relevant information and creatively generate answers based on that information.
Why is Retrieval Augmented Generation Special?
The real magic of RAG lies in its ability to pull in up-to-date, highly relevant information before generating a response.
Unlike traditional models that rely solely on their pre-trained knowledge (imagine an author writing from what they already know without doing new research), RAG actively seeks out the latest information, ensuring that the answers you get are not just accurate but also as current as possible.
RAG’s Broader Impact
By integrating the ability to retrieve and generate information, Retrieval Augmented Generation systems are not just responding, they are learning and adapting with each interaction.
This makes them incredibly powerful tools for applications where staying current is crucial—like news reporting, medical advice, or dynamic educational tools where information changes rapidly.
Retrieval Augmented Generation tools like ChatGPT represent a significant step forward in making AI interactions more meaningful and informed.
Learn More: Top 7 Must-Know Machine Learning Tools
How Retrieval Augmented Generation (RAG) Works?
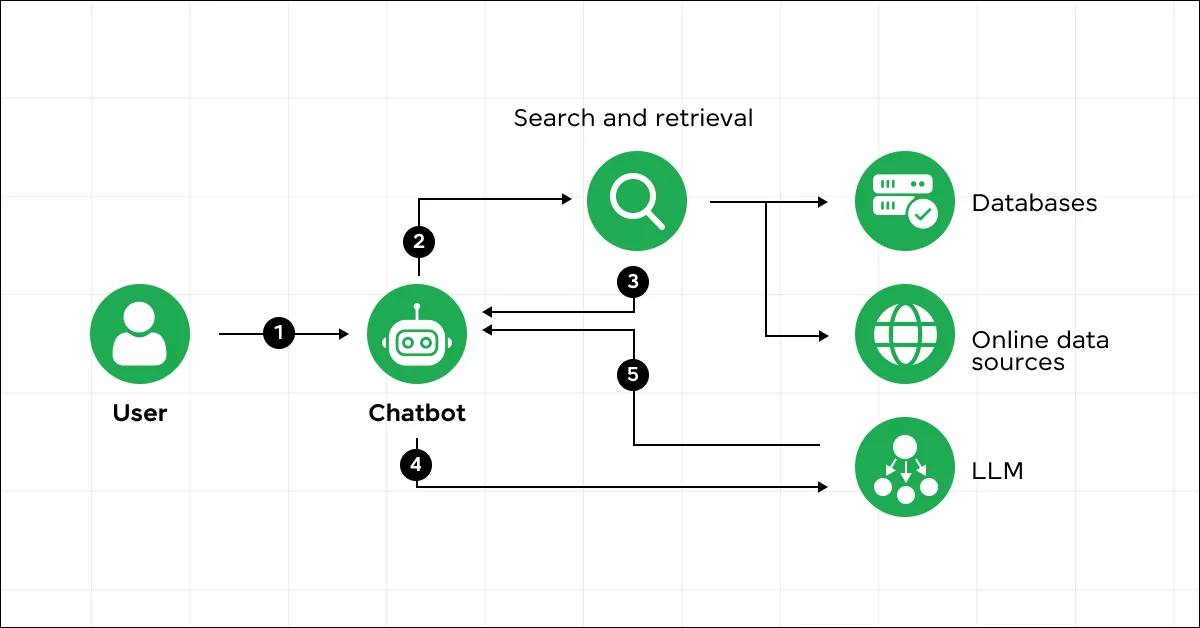
Now that you understand what Retrieval Augmented Generation is, let us move to the next section of our article where we will understand how it works.
Before we go, make sure that you know the basics of machine learning cracked down. If not, consider enrolling for a certified online Artificial Intelligence & Machine Learning course offered by a professional institution as that can help you strengthen your basics as well as provide you with an industry-grade certificate.
Let us now understand the workings of Retrieval Augmented Generation:
Step-by-Step Breakdown of RAG:
- Understanding the Task at Hand (Query Processing)
- Retrieval Augmented Generation starts with what is called ‘query processing.’ This means when you ask RAG a question or give it a prompt, it first needs to understand exactly what you’re asking.
- Finding the Right Data (Information Retrieval)
- Next, Retrieval Augmented Generation needs to find the right information, much like how you would search for recipes. Using advanced algorithms, RAG scours through vast digital databases to find data that best matches your query. This step is crucial because the information it pulls up will form the basis of the answer RAG will generate.
- Generating the Content
- Finally, RAG takes the information it has gathered and starts generating an answer. This part of the process involves synthesizing all the retrieved information to produce a coherent and contextually appropriate response.
Also Read: Exploring the Influence of AI and Machine Learning in Full Stack Development
Why is Each Step Important?
- Query Processing: This step ensures that RAG fully understands what information you are seeking. Just as important as choosing the right dish to satisfy your hunger, correctly interpreting the query is essential for retrieving the right information.
- Information Retrieval: This is all about accuracy and relevance. If you get this wrong, just like using salt instead of sugar in a cake, the outcome will be disappointing. RAG aims to retrieve the most relevant and precise information to make sure the final answer is on point.
- Content Generation: This is where creativity comes into play. RAG combines all the retrieved data to generate an answer that not only addresses the query but does so in a way that is easy to understand and appropriate for the context. It’s like adding your personal touch to the meal to make it special.
Retrieval Augmented Generation’s Interaction with Data
It’s important to note that RAG dynamically interacts with the information. Unlike a static database that remains unchanged, the sources RAG taps into can be updated, and RAG’s ability to access the most current data makes it incredibly powerful.
This makes RAG a robust tool in the world of AI, turning it into a knowledgeable assistant who’s always ready to help you with accurate and up-to-date information.
Explore: The Rise (RAISE) Of Artificial Intelligence
Practical Applications of Retrieval Augmented Generation (RAG)
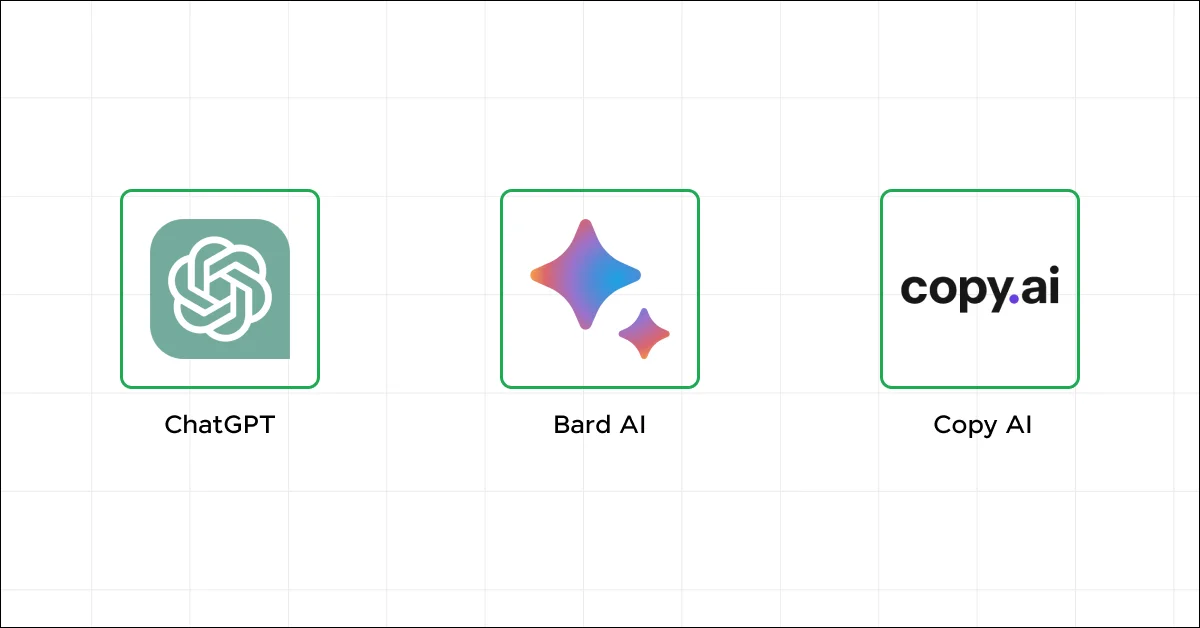
Understanding the technical workings of Retrieval Augmented Generation (RAG) is fascinating, but seeing how it applies to everyday situations can truly highlight its value.
RAG’s ability to fetch and generate information dynamically makes it incredibly versatile across various fields.
Let’s explore how Retrieval Augmented Generation could directly impact your life through its diverse applications.
1. Enhancing Educational Tools
If you’re a student or a lifelong learner, imagine having a tutor who not only knows the entirety of your course materials but can also pull in the most recent studies, examples, and data to answer your questions.
RAG can power educational platforms that provide personalized learning experiences. Whether you’re struggling with quantum mechanics or Renaissance art, RAG-enhanced tools can retrieve academic papers, educational videos, and contextual information to tailor explanations to your specific needs.
Also, see about the trending AI Tools for Students
2. Revolutionizing Customer Support
Have you ever been frustrated with customer support that doesn’t seem to understand your problem? Retrieval Augmented Generation could change that.
By integrating RAG into customer service chatbots, companies can provide you with more accurate, informed responses.
These bots can pull information from product manuals, customer history, and frequently asked questions to offer solutions that are not just generic but specifically suited to your issue and past interactions.
Also Read: The Influence of Chatbots on Customer Services: Benefits and Impact
3. Transforming Content Creation
For writers, journalists, and creators, RAG could be like having a research assistant who’s always on duty. When writing an article or preparing a report, RAG can quickly gather the latest data, cite relevant sources, and even suggest content improvements based on current trends.
This not only speeds up the creative process but also enhances the quality of content with up-to-date and comprehensive information.
Also, Learn about the Top 9 AI Tools for Content Creation
4. Optimizing Legal and Medical Research
Professionals in fields like law and medicine often spend countless hours digging through case files and medical journals.
RAG could streamline this process by instantly retrieving necessary documents and studies, drawing from the latest legal precedents or medical research to provide practitioners with timely, relevant information. This means better, faster decision-making in critical fields that impact lives.
5. Improving Accessibility of Information
For individuals with disabilities, especially those with visual impairments or learning disabilities, RAG can offer more accessible ways to interact with large volumes of text.
By providing spoken-word responses or simplified summaries of complex materials, RAG can make information more accessible to everyone, regardless of their ability to read or interact with traditional text.
Must Find Out Top 10 Machine Learning Applications You Should Know
Why These Applications Matter?
The applications of Retrieval Augmented Generation extend beyond convenience; they reach into enhancing efficiency, accessibility, and personalization.
For you, this means interacting with technology that not only understands your needs better but also responds in a way that feels more intuitive and helpful.
As we continue to develop and integrate these systems, the potential for more innovative applications will only grow, making technology an even more indispensable part of our everyday experiences.
Read More: 30+ Generative AI Terms That You Must Know
Challenges and Considerations of Retrieval Augmented Generation
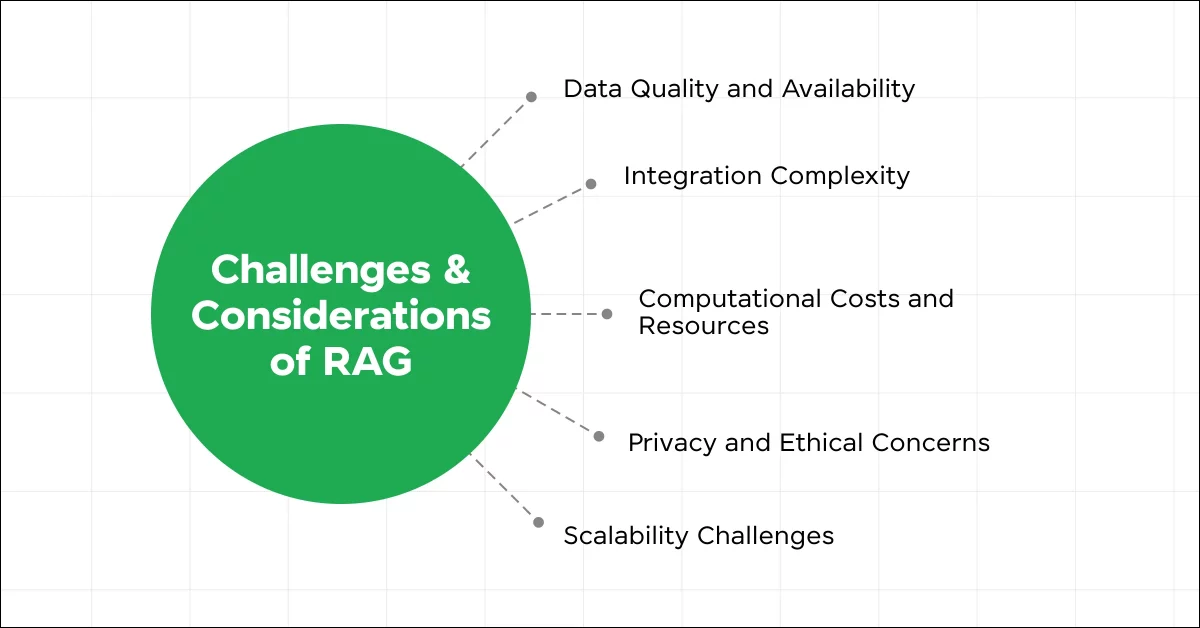
While Retrieval Augmented Generation (RAG) offers remarkable capabilities, as with any technology, it comes with its own set of challenges and considerations.
These can impact how effectively it can be implemented and used in real-world applications. Let’s delve into some of these challenges and what they mean for you.
1. Data Quality and Availability
Imagine you’re trying to cook a healthy meal, but all you have are some old spices and expired ingredients. The quality of your meal would naturally suffer.
Similarly, RAG systems rely heavily on the quality and availability of the data they access. If the information retrieved is outdated, incomplete, or biased, the responses generated will be less accurate and potentially misleading.
Ensuring that the data is comprehensive, current, and unbiased is crucial, but this can be a significant challenge given the vast amounts of data RAG needs to operate effectively.
Also Explore: Top 8 Big Data Visualization Tools That You Should Know in 2025
2. Integration Complexity
Integrating Retrieval Augmented Generation into existing systems isn’t as simple as plugging in a new appliance. It’s more like rewiring your home’s electricity—it requires careful planning, execution, and testing.
The process involves merging RAG with different software environments and needs, which can be complex and time-consuming.
3. Computational Costs and Resources
Running RAG models involves significant computational power and resources. For organizations, this can mean higher operational costs as more powerful hardware or cloud services might be necessary to handle the workload.
For you as a user, this could translate into slower response times or higher costs for services that use RAG technology, especially in its initial stages or complex applications.
Read: Top 6 Essential Prerequisites For Machine Learning
4. Privacy and Ethical Concerns
Privacy is a major concern with technologies like RAG that access and generate information from vast data pools.
For instance, if you’re using a RAG-powered health advisor, you’d want to be sure that your personal health data isn’t being misused or inadvertently exposed.
Ensuring that RAG systems adhere to privacy laws and ethical standards is very important to protect your information and maintain trust in the technology.
5. Scalability Challenges
As more people and industries begin to use RAG, scaling the technology to handle increasing demands while maintaining performance will be a challenge.
Think of it like a local restaurant that suddenly becomes a nationwide hit. Scaling up to meet demand without compromising on quality or speed is a significant hurdle for Retrieval Augmented Generation.
If you want to learn more about Retrieval Augmented Generation, then consider enrolling in GUVI’s Certified Artificial Intelligence & Machine Learning Course which not only gives you theoretical knowledge but also practical knowledge with the help of real-world projects.
Also Read: Top 10 Steps to Kickstart Your Career as a Machine Learning Engineer
Conclusion
In conclusion, Retrieval Augmented Generation (RAG) represents a significant advancement in artificial intelligence and machine learning, blending the power of retrieval-based and generative models to create systems that are more informed, responsive, and adaptive.
As RAG continues to evolve, it promises to enhance our daily applications, making our interactions with AI deeply personalized and contextually relevant. Despite facing challenges, the future of RAG is promising, aiming to integrate more seamlessly into our daily lives and improve decision-making.
As we look forward, the potential of RAG to enrich and simplify our digital interactions is boundless, leading to a new era of intelligent and interactive technology.
Must Explore: Top 9 Machine Learning Project Ideas For All Levels [with Source Code]
FAQs
1. Can RAG be used to generate content in multiple languages?
Yes, RAG can be adapted to work with multiple languages as long as there is sufficient data in those languages to support both the retrieval and generation processes.
2. What industries could benefit the most from RAG?
Industries like education, customer service, healthcare, legal, and content creation stand to gain significantly from the enhanced capabilities of RAG.
3. Is there a risk of data bias in RAG systems?
Yes, like any AI system, RAG can be susceptible to data biases present in its training datasets. Continuous monitoring and updating of data sources are necessary to mitigate this risk.
4. Can RAG technology be integrated into existing AI systems?
Yes, RAG can be integrated into existing AI systems, although this might require significant modifications to accommodate the retrieval component.
5. Can RAG work offline?
Working offline is challenging for RAG systems as they depend heavily on accessing large online databases for information retrieval.
Did you enjoy this article?